Artificial Intelligence for Predicting Primary Antegrade Wiring Success of Chronic Total Occlusion Crossing
© 2024 HMP Global. All Rights Reserved.
Any views and opinions expressed are those of the author(s) and/or participants and do not necessarily reflect the views, policy, or position of the Journal of Invasive Cardiology or HMP Global, their employees, and affiliates.
J INVASIVE CARDIOL 2024. doi:10.25270/jic/24.00217. Epub September 30, 2024.
Abstract
Background. Incorporating artificial intelligence (AI)-based clinical decision support in the cardiac catheterization laboratory could revolutionize percutaneous coronary intervention (PCI) and interventional cardiology (IC).
Methods. The authors conducted a survey to assess the estimates of ICs regarding primary antegrade wiring (AW) crossing success, on a scale of 0% to 100%, for 15 chronic total occlusion (CTO) PCIs and compare them with estimates from a machine learning model (MLM). For the AI-based prediction, the authors used a MLM with an area under the curve of 0.78. The primary endpoint was the difference between AI-generated and human estimates.
Results. A total of 130 ICs participated in the survey. The mean participant age was 51.7 ± 10.6 years. The median difference between the AI and the human prediction was less than 20% for 7 cases, 20% to 30% for 6 cases, and greater than 30% for 2 cases. Successful AW-based crossing by an experienced CTO operator was achieved in 8 of 15 cases. The operator predictions aligned with the real-life outcomes in 9 of 15 cases (60.0%), while AI correctly predicted the success/failure of primary AW CTO crossing in 11 of 15 cases (73.3%). Operators with more experience (> 20 CTO PCIs annually) predicted higher success than less experienced operators for 5 cases. Operators who train dedicated advanced fellows predicted higher success in 6 cases and showed worse alignment with the AI prediction in 4 cases.
Conclusions. The study shows that an MLM could assist CTO PCI planning by improving the accuracy of primary AW success predictions.
Introduction
Incorporating artificial intelligence (AI)-based clinical decision support in the cardiac catheterization laboratory has the potential to revolutionize interventional cardiology (IC)1. According to a recent survey, 63.7% of interventional cardiologists (ICs) expressed optimism about the integration of AI in IC, while 60.6% anticipated the implementation of AI in their personal clinical practice within 5 years.2
There is growing interest in applying machine learning to predict outcomes of chronic total occlusion (CTO) percutaneous coronary intervention (PCI) and guide intraprocedural decisions.3-5 Decision-making regarding crossing strategies in CTO PCI is highly variable and dependent on operator experience.6 Antegrade wiring (AW) is the most commonly used CTO crossing strategy, with increasing success in recent years (2016: 46%; 2021: 61%).7 In this study, we compared the predictions for successful AW of CTO lesions made by ICs with those from a machine learning model (MLM).8
Methods
The survey questionnaire was collaboratively developed by the co-authors through an interactive process. The final survey included 18 questions focusing on demographics and operator experience, along with 30 questions regarding the procedural strategies and success of AW in 15 cases of CTO PCI. The 15 cases were selected by a panel of 3 ICs experienced in CTO PCI. These cases were chosen to represent a wide spectrum of complexity in CTO lesions, including variations in lesion length, calcification, vessel tortuosity, and prior intervention. The selection aimed to provide a realistic and diverse set of cases commonly encountered in clinical practice. These 15 CTO PCIs were performed by an experienced CTO PCI operator at a large tertiary hospital. For each case, operators were presented a baseline coronary angiogram video and received information about the CTO target vessel location (left anterior descending artery [LAD], left circumflex artery [LCX], right coronary artery [RCA], left main artery [LM], or obtuse marginal artery [OM]) and any previous attempts (yes or no). They were then asked 2 questions per case: (1) “Please estimate the probability of successfully crossing the CTO lesion using primary AW in case” and (2) “Do you predict successful crossing of the CTO lesion with AW?”. The participants estimated the probability on a scale of 0% to 100%. The survey was conducted using REDCap (Research Electronic Data Capture)9,10 and was distributed to IC attendings and fellows via social media and email lists. The survey was distributed via email to the list of the Cardiovascular Innovations Foundation and through relevant social media platforms, specifically X (formerly known as Twitter), where many ICs engage professionally. The survey was available for a period of 7 weeks. The study was approved by the institutional review board of the Minneapolis Heart Institute.
For the AI-based prediction of primary AW success, we used a recently developed MLM, which is accessible online for public use at https://www.progresscto.org/predict-aw-success.8 The model incorporates 14 variables and has high predictive capacity for successful primary AW in CTO PCI, with an area under the curve (AUC) of 0.780. The angiograms were assessed by 2 ICs and their angiographic characteristics are reported in Table 1. These characteristics, along with data on any previous attempts, were inputted into the MLM for generating the AI prediction. The angiograms can be viewed through the following link: https://redcap.link/09zqhj8m. The primary endpoint was the difference between AI-generated predictions and human estimates.
Categorical variables were expressed as percentages and compared using the Pearson’s chi-square test. Continuous variables are presented as mean ± SD or as median (interquartile range [IQR]), unless otherwise specified, and were compared using the independent-samples t-test for normally distributed variables and the Mann-Whitney U test for non-parametric variables, as appropriate. All statistical analyses were performed using R Statistical Software, version 4.2.2 (R Foundation for Statistical Computing). A P-value of less than 0.05 was considered statistically significant.
Results
Participants’ demographics
A total of 130 ICs (125 attendings/consultants and 5 fellows/trainees) participated in the survey (Figure 1). The mean participant age was 51.7 ± 10.6 years. Among respondents, 3.1% were women. Most participants practiced in the United States (63.8%), followed by Europe (9.2%) and India (6.2%). They worked at academic institutions (38.8%), community hospitals with academic affiliation (22.5%), community hospitals (17.8%), private hospitals (12.4%), Veterans Affairs (VA) hospitals (2.3%), and government hospitals (6.2%) (Table 2).
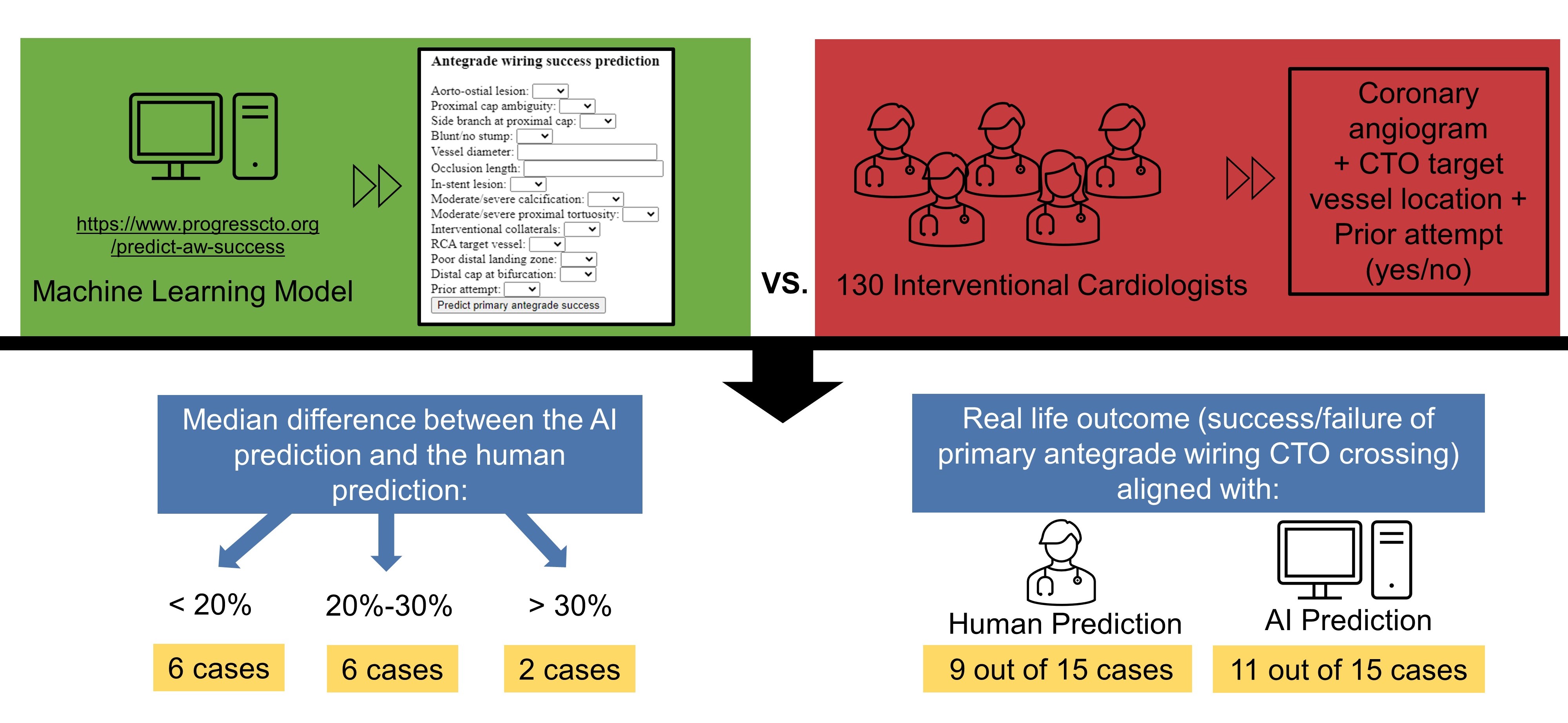
AI and ICs predictions
The distribution of the predictions of the ICs and the respective prediction of the AI model are presented in Figure 2. There was significant discrepancy between the operator and the AI predictions: the median difference in estimated success rates between the AI prediction and the human prediction was less than 20% for 6 cases (cases 1, 3, 4, 9, 11, and 13); 20% to 30% for 6 cases (cases 2, 6, 7, 12, 14, and 15); and greater than 30% for 2 cases (cases 8 and 10). The cutoffs were selected a priori based on expert consensus, with the goal of capturing clinically meaningful differences in predictions. However, we also performed an exploratory analysis using alternative cutoffs, and the results remained consistent with the primary analysis.
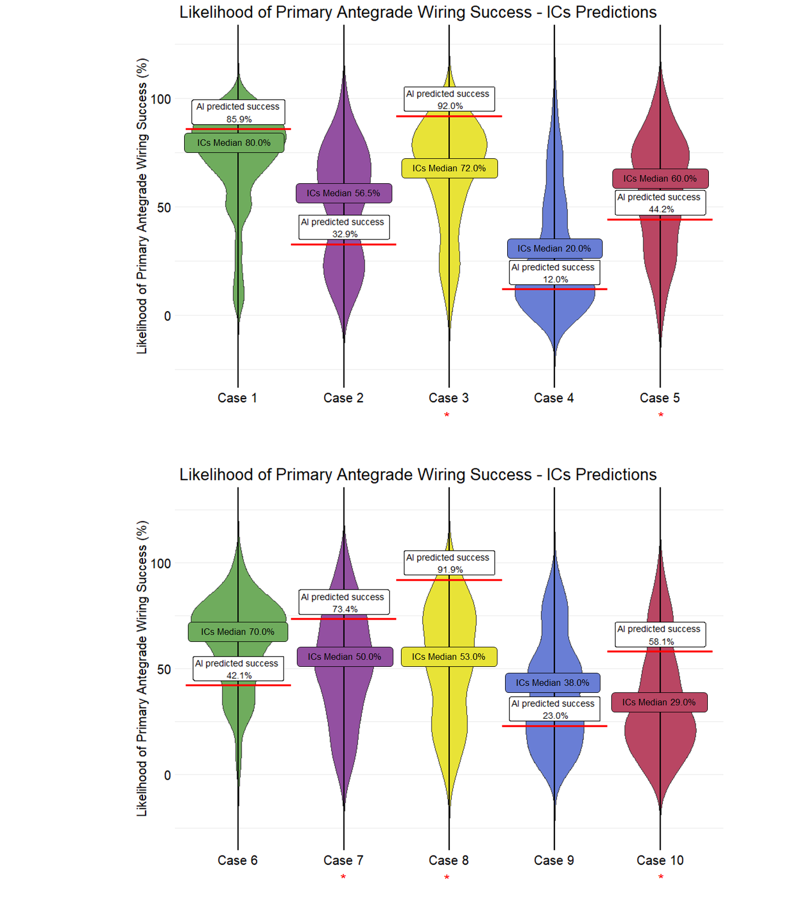
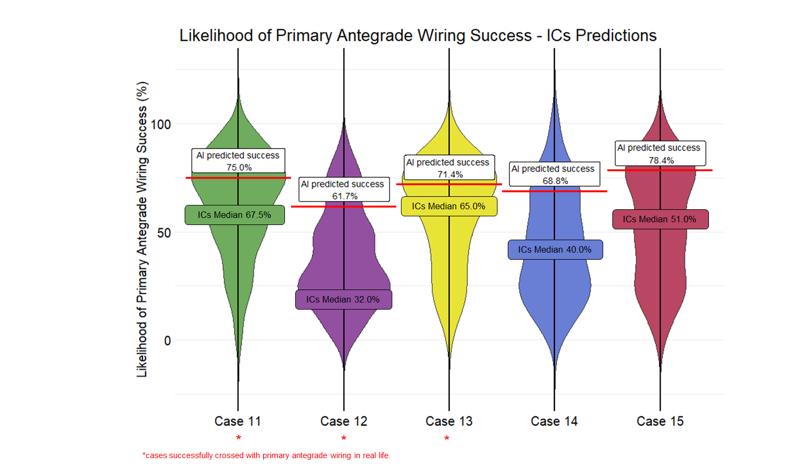
Crossing with primary AW was successful in 8 cases (cases 3, 5, 7, 8, 10, 11, 12, and 13). If the median ICs prediction or the AI prediction was equal to or greater than 50%, their prediction was considered a success with primary AW. Using this threshold, the operator predictions aligned with the outcomes in 9 of the 15 cases (60.0%), while AI correctly predicted the success/failure of primary AW CTO crossing in 11 of the 15 cases (73.3%). With a threshold of 60%, the ICs correctly predicted 9 cases, and the AI correctly predicted 10 cases, while with a higher threshold of 70%, the ICs correctly predicted 6 cases, and the AI correctly predicted 10 cases.
Operators highly comfortable with antegrade dissection re-entry (ADR) (46.4% with comfort level > 80%) predicted similar success rates as those less comfortable with ADR (≤ 80% comfort level) in 14 of the 15 cases. However, for case 2, the more comfortable group had a higher success rate for primary AW (63.5% vs 50.0%, P < .001). Similarly, operators with high comfort in retrograde techniques (32.0% with > 80% comfort level) and their less comfortable counterparts (≤ 80% comfort level) had comparable success rates in 14 of the 15 cases.
Operators who do CTO proctoring (73.6%) predicted higher success for 3 cases (case 2: 67.5% vs 50.0%, P = .006; case 3: 80.0% vs 70.0%, P = .004; case 15: 74.0% vs 50.0%, P = .003), compared with operators who do not do CTO proctoring. However, they showed better alignment with AI predictions for only 1 case, case 3, for which the median difference was 12.0% for the operators who do CTO proctoring vs 22.0% for those who do not (P = .019).
Operators practicing in the US (63.8%) had significant differences in their predictions compared with non-US operators in 9 cases: case 4 (21.1% vs 37.5%, P < .001), case 5 (51.6% vs 64.7%, P = .005), case 6 (60.2% vs 67.6%, P = .051), case 7 (46.3% vs 61.4%, P = .002), case 9 (32.8% vs 50.0%, P < .001), case 10 (29.3% vs 45.3%, P = .001), case 11 (59.6% vs 68.6%, P = .043), case 12 (34.6% vs 44.2%, P = .029), and case 15 (49.8% vs 59.5%, P = .057). The US operators showed better alignment with the AI predictions for 4 cases and worse alignment for 1 case compared with the non-US operators. The median differences between the AI prediction and the IC prediction for these cases were as follows: case 4: 9.5% vs 18.0%, P = .006; case 5: 19.2% vs 28.9%, P = .003; case 6: 23.9% vs 28.4%, P = .022; case 9: 13.0% vs 27.0%, P < .001; case 10: 33.1% vs 21.9%, P = .013.
Operators with more experience (69%; performing > 20 CTO PCIs annually) (Figure 3), predicted higher success with primary AW for 5 cases (cases 1, 2, 6, 11, and 13) compared with less experienced operators. The median difference between the AI prediction and the human estimates was higher for experienced operators for case 2 (27.1% vs 22.9%, P = .044) and lower for case 13 (10.2% vs 17.3%, P = .005). Comparing the estimates between IC fellows (n = 5) and attendings (n = 125), no statistically significant differences were detected in any case except for case 7, where fellows predicted a higher success rate (80.0% vs. 50.0%, P = .034).
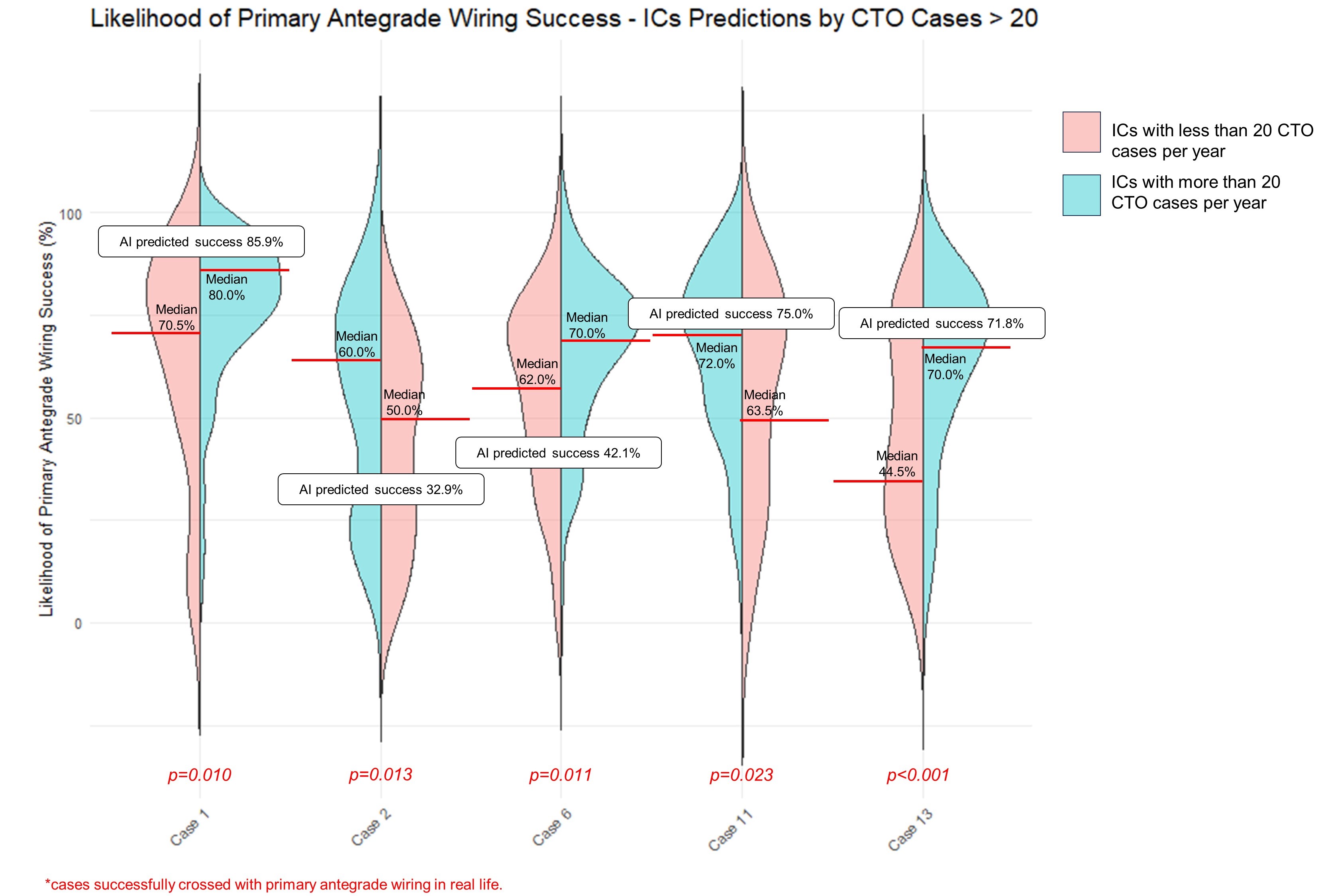
Operators who train dedicated complex and high-risk coronary intervention (CHIP)/CTO fellows (ie, advanced fellows, beyond basic interventional cardiology training) (23.3%) predicted higher primary AW success for 6 cases (cases 2, 3, 6, 9, 13, and 15) compared with operators who do not train dedicated CHIP/CTO fellows. The median differences between the AI prediction and the human estimates were higher for operators who train dedicated CHIP/CTO fellows for case 2 (31.1% vs 22.1%, P = .019), case 4 (13.0% vs 10.0%, P = .032), case 5 (30.9% vs 22.15%, P = .037), and case 6 (35.9% vs 27.9%, P = .043).
Discussion
To the best of our knowledge, this is the first study comparing operator predictions with AI predictions in CTO PCI. The main findings were (1) significant variability in the predictions of ICs on primary AW success for CTO crossing, with more experienced operators predicting higher success than less experienced operators; and (2) the AI model correctly predicted the real-life outcomes (primary AW success or failure) in 11 of the 15 cases, slightly surpassing the predictions made by ICs, who were aligned with the outcome in 9 of the 15 cases.
The selection of a primary crossing strategy in CTO PCI depends on several factors, such as proximal cap ambiguity, poor distal vessel quality, presence of side branch, calcification, lesion length, and presence of interventional collaterals.6 Use of a machine learning prediction model can fit into the existing clinical workflow and improve the efficiency of the pre-procedural planning, while taking into consideration interactions that are more complex and maybe invisible to the human eye. The model can not only provide the likelihood of successful crossing with primary AW, but also report how much each characteristic influenced the outcome, either increasing or decreasing the likelihood of success.8
How can the high discrepancy (> 30%) between the AI and ICs' predictions (case 8 and 10) be explained? Case 10 was an RCA CTO with no prior attempt, while case 8 was an LM CTO lesion with a prior failed attempt. A potential explanation for the high difference of predictions in case 8 could be that LM CTOs are infrequent (estimated prevalence of 0.04%)11 and operators/AI may be less comfortable making predictions in such rare scenarios. Case 10 involved an in-stent lesion (Table 1), which was the primary factor that influenced the likelihood of success in the AI model. In-stent CTOs account for approximately 15% of all CTO PCIs, are more likely to be crossed successfully with AW, and have similar success rates as de novo lesions.12 The real-life outcome was successful crossing, which was aligned with the AI, but not the human, prediction.
Limitations
The utilization of email lists and social media for participant recruitment may have introduced selection bias. Participants might be more interested in the topic of the survey than non-responders, which might also result in selection bias. Additionally, the level of expertise reported by the participants was self-assessed, which may not accurately reflect their actual proficiency. Finally, we assessed IC vs AI prediction in only 15 cases, and a larger sample size is warranted in future studies.
Conclusions
The findings of our study suggest that an MLM could improve predictions of primary AW success for CTO PCI, thereby facilitating procedural planning.
Affiliations and Disclosures
Michaella Alexandrou, MD1; Athanasios Rempakos, MD1; Deniz Mutlu, MD1; Dimitrios Strepkos, MD1; Pedro E. P. Carvalho, MD1; Ahmed Al Ogaili, MD1; Ali Bahbah, MD1; Anastasios Milkas, MD2; Ioannis Tsiafoutis, MD3; Khaldoon Alaswad, MD4; Mir Babar Basir, DO4; Rhian Davies, DO, MS5; Farouc A. Jaffer, MD, PhD6; William Nicholson, MD7; Lorenzo Azzalini, MD, PhD, MSc8; Sevket Gorgulu, MD9; Jaikirshan J. Khatri, MD10; Sripal Bangalore, MD11; Bavana V. Rangan, BDS, MPH1; Olga C. Mastrodemos, BA1; M. Nicholas Burke, MD1; Yader Sandoval, MD1; Emmanouil S. Brilakis, MD, PhD1
From the 1Minneapolis Heart Institute and Minneapolis Heart Institute Foundation, Abbott Northwestern Hospital, Minneapolis, Minnesota, USA; 2Athens Naval and Veterans Hospital, Athens, Greece; 3Red Cross Hospital of Athens, Athens, Greece; 4Henry Ford Cardiovascular Division, Detroit, Michigan, USA; 5WellSpan York Hospital, York, Pennsylvania, USA; 6Massachusetts General Hospital, Boston, Massachusetts, USA; 7Emory University Hospital Midtown, Atlanta, Georgia, USA; 8Division of Cardiology, Department of Medicine, University of Washington, Seattle, Washington, USA; 9Biruni University Medical School, Istanbul, Turkey; 10Cleveland Clinic, Cleveland, Ohio, USA; 11Division of Cardiology, New York University, New York, USA.
Acknowledgments: The authors are grateful for the philanthropic support of our generous anonymous donors (2), and the philanthropic support of Drs Mary Ann and Donald A. Sens, Mr Raymond Ames and Ms Barbara Thorndike, the Frank J. and Eleanor A. Maslowski Charitable Trust, the Joseph F. and Mary M. Fleischhacker Family Foundation, Mrs Diane and Dr Cline Hickok, Mrs Marilyn and Mr William Ryerse, Mr Greg and Mrs Rhoda Olsen, Mrs Wilma and Mr Dale Johnson, the Mrs Charlotte and Mr Jerry Golinvaux Family Fund, the Roehl Family Foundation, and the Joseph Durda Foundation. The generous gifts of these donors to the Minneapolis Heart Institute Foundation’s Science Center for Coronary Artery Disease (CCAD) helped support this research project.
Disclosures: Dr Alaswad is a consultant and speaker for Boston Scientific, Abbott Cardiovascular, Teleflex, and Cardiovascular Systems Inc. Dr Basir is a consultant for Abbott Vascular, Abiomed, Cardiovascular Systems, Inc (CSI), Chiesi, and Zoll. Dr Davies receives speaking honoraria from Abiomed, Asahi Intecc, Boston Scientific, Medtronic, Teleflex, and Shockwave Medical; she also serves on advisory boards for Abiomed, Avinger, Boston Scientific, Medtronic, and Rampart. Dr Jaffer has done sponsored research for Canon, Siemens, Shockwave, Teleflex, Mercator, and Boston Scientific; has been a consultant for Boston Scientific, Siemens, Magenta Medical, IMDS, Asahi Intecc, Biotronik, Philips, and Intravascular Imaging Inc.; has equity interest in Intravascular Imaging Inc. and DurVena; and has the right to receive royalties through Massachusetts General Hospital licensing arrangements with Terumo, Canon, and Spectrawave. Dr Azzalini has received consulting fees from Teleflex, Abiomed, GE Healthcare, Asahi Intecc, Philips, Abbott Vascular, Reflow Medical, and Cardiovascular Systems, Inc. Dr Khatri has received personal honoraria for proctoring and speaking from Abbott Vascular, Medtronic, Terumo, Shockwave, and Boston Scientific. Dr Bangalore serves on the advisory boards for Abbott Vascular, Boston Scientific, Biotronik, Amgen, Pfizer, Merck, REATA, Inari, and Truvic. Dr Burke receives consulting and speaker honoraria from Abbott Vascular and Boston Scientific. Dr Sandoval receives consulting/speaker honoraria from Abbott Diagnostics, Roche Diagnostics, Zoll, Phillips; is an associate editor for JACC Advances; and holds patent 20210401347. Dr Brilakis receives consulting/speaker honoraria from Abbott Vascular, the American Heart Association (associate editor, Circulation), Amgen, Asahi Intecc, Biotronik, Boston Scientific, Cardiovascular Innovations Foundation (Board of Directors), CSI, Elsevier, GE Healthcare, IMDS, Medicure, Medtronic, Siemens, Teleflex, and Terumo; receives research support from Boston Scientific and GE Healthcare; is the owner of Hippocrates LLC; and is a shareholder in MHI Ventures, Cleerly Health, and Stallion Medical. The remaining authors report no financial relationships or conflicts of interest regarding the content herein.
Address for correspondence: Emmanouil S. Brilakis, MD, PhD, Minneapolis Heart Institute, 920 E 28th Street #300, Minneapolis, MN 55407, USA. Email: esbrilakis@gmail.com; X: @CCAD_MHIF, @esbrilakis, @m1chaella_alex
References
1. Samant S, Bakhos JJ, Wu W, et al. Artificial intelligence, computational simulations, and extended reality in cardiovascular interventions. JACC Cardiovasc Interv. 2023;16(20):2479-2497. doi:10.1016/j.jcin.2023.07.022
2. Alexandrou M, Rempakos A, Mutlu D, et al. Interventional cardiologists' perspectives and knowledge towards artificial intelligence. J Invasive Cardiol. 2024;36(8). doi:10.25270/jic/24.00052
3. Nakachi T, Yamane M, Kishi K, et al. Machine learning for prediction of technical results of percutaneous coronary intervention for chronic total occlusion. J Clin Med. 2023;12(10):3354. doi:10.3390/jcm12103354
4. Zhou Z, Gao Y, Zhang W, et al. Deep learning–based prediction of percutaneous recanalization in chronic total occlusion using coronary CT angiography. Radiology. 2023;309(2):e231149. doi:10.1148/radiol.231149
5. Liu X, Du T, Zhang H, Sun C. Detection and classification of chronic total occlusion lesions using deep learning. Annu Int Conf IEEE Eng Med Biol Soc. 2019;2019:828-831. doi:10.1109/EMBC.2019.8856696
6. Wu EB, Brilakis ES, Mashayekhi K, et al. Global chronic total occlusion crossing algorithm: JACC state-of-the-art review. J Am Coll Cardiol. 2021;78(8):840-853. doi:10.1016/j.jacc.2021.05.055
7. Kostantinis S, Simsek B, Karacsonyi J, et al. In-hospital outcomes and temporal trends of percutaneous coronary interventions for chronic total occlusion. EuroIntervention. 2022;18(11):e929-e932. doi:10.4244/EIJ-D-22-00599
8. Rempakos A, Alexandrou M, Mutlu D, et al. Predicting successful chronic total occlusion crossing with primary antegrade wiring using machine learning. JACC Cardiovasc Interv. 2024;17(14):1707-1716. doi:10.1016/j.jcin.2024.04.04
9. Harris PA, Taylor R, Minor BL, et al; REDCap Consortium. The REDCap consortium: building an international community of software platform partners. J Biomed Inform. 2019;95:103208. doi:10.1016/j.jbi.2019.103208
10. Harris PA, Taylor R, Thielke R, Payne J, Gonzalez N, Conde JG. Research electronic data capture (REDCap)--a metadata-driven methodology and workflow process for providing translational research informatics support. J Biomed Inform. 2009;42(2):377-381. doi:10.1016/j.jbi.2008.08.010
11. Xenogiannis I, Karmpaliotis D, Alaswad K, et al. Left main chronic total occlusion percutaneous coronary intervention: a case series. J Invasive Cardiol. 2019;31(7):E220-E225.
12. Vemmou E, Quadros AS, Dens JA, et al. In-stent CTO percutaneous coronary intervention: individual patient data pooled analysis of 4 multicenter registries. JACC Cardiovasc Interv. 2021;14(12):1308-1319. doi:10.1016/j.jcin.2021.04.003