Traceability in Artificial Intelligence: A Critical Look at Platforms in Dermatology
© 2025 HMP Global. All Rights Reserved.
Any views and opinions expressed are those of the author(s) and/or participants and do not necessarily reflect the views, policy, or position of The Dermatologist or HMP Global, their employees, and affiliates.
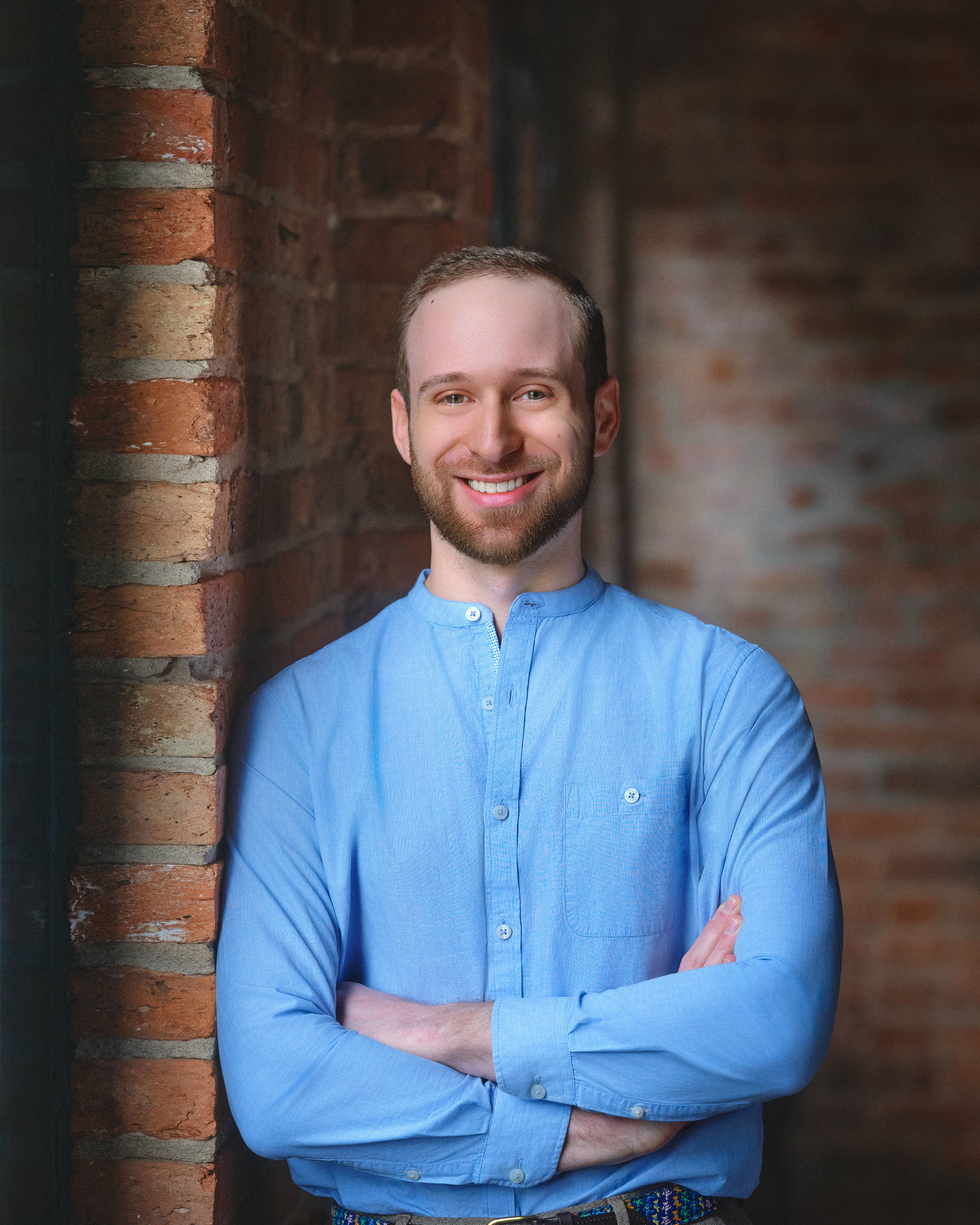
Products pitching artificial intelligence (AI)-based clinical solutions for a variety of clinical dermatology use cases have rapidly proliferated in recent years. Perhaps the most well-known use cases involve AI-powered image classification in the context of macroscopic diagnosis. The foundational concern with this type of AI tool is establishing the tool’s precision or how closely it reflects the true biologic condition of the patient. As of 2024, there are several AI-powered smartphone applications intended to classify skin lesions that are not US Food and Drug Administration (FDA) approved, along with an FDA-approved medical device. These platforms should not be confused with teledermatology or teledermoscopy platforms, which transmit images or data to remote dermatologists for evaluation.
These first-generation products already have a concerning track record of adverse events, from near misses to sentinel events. A particularly high-profile example was the Epic Sepsis Model. Epic trained the AI model on a limited data set across 3 hospital systems. In addition, the proprietary nature of the model precluded disclosure of performance metrics of predictive output compared to gold standards. There were 2 major issues with this setup: the limited data created a model that was ill-prepared to recognize problems in real-world settings and the lack of visibility across the system’s performance prevented physicians from recognizing the model’s flaws and correcting their treatments. A 2021 external validation found that the model failed to flag 67% of cases of impending sepsis, despite being implemented across dozens of hospital systems.1 Patient safety experts have suggested that reliable, reproducible traceability standards should include core metadata that would allow clinicians, regulators, and industry professionals to conduct adequate post-market surveillance of failure incidents, model bias, adverse events, and inequitable health outcomes.2
AI-Powered Tools vs Traditional Diagnostic Tests
To delve into this question from the context of an AI-powered tool, it is essential to consider how these tools differ from traditional diagnostic tests. First, consider the methodological foundations of a traditional lab test such as a serum assay for vitamin D levels. The test is performed using liquid chromatographytandem mass spectrometry or high-performance liquid chromatography, methods that are meticulously validated for accuracy. Standardized reference materials, such as those provided by the National Institute of Standards and Technology, ensure consistency across laboratories worldwide. The test input reflects the physical reality of the specimen, such as how much 25-hydroxy vitamin D is present in the sample. The output is a numerical quantification of this reality, and the “levers” of normal vs abnormal can be transparently adjusted by changing the sensitivity and specificity thresholds.
In contrast, an AI-powered tool relies on machine-learning (ML) models that are trained using pattern recognition systems on large datasets (input) to make probabilistic predictions (output). In the case of image recognition devices and platforms, the algorithms may consist of supervised, unsupervised, or neural network layers in array. To produce reliable predictions, these devices need substantial and diverse datasets free of bias. Assembling a reliable data set for training is much trickier than it may appear on the surface. What if the training or annotation repertoire contains errors, ambiguities, or misleading assumptions? For example, pigmented lesions that straddle the diagnostic line between melanoma and severely dysplastic nevus introduce significant variability. Board-certified dermatopathologist Dr Buu T. Duong comments, “Given the same complex melanocytic lesion, it is not uncommon for multiple dermatopathologists to render differing opinions.” A 2017 study in the British Medical Journal found a concordance of only 82% for pathologic assessment of atypia in pigmented lesions.3 If the histopathologic diagnoses used to label training images are themselves contentious, the resulting AI model inherits these ambiguities.
The Importance of Traceability
The path to making safer and more reliable AI-powered tools is analogous to validation of any experimental outcome in science: reproducible results that reflect the nature of reality. In the context of ML platforms, this process is encapsulated in traceability. In its most generic sense, traceability is the overarching map of every element in the software: the datasets, the code, the roadway for how data move through the software, and how decision-making is determined. Traceability should also account for potential system changes. In an ideal world, if an issue is identified in the software, traceability should allow the software engineer to trace the issue back to a source and initiate a change in the system.
From a clinical perspective, providing traceability helps users trust and verify an algorithm’s conclusions, from input to output. In the context of dermatology, this includes transparency around the datasets used for training, the features prioritized by the model, and the reasoning behind its diagnostic recommendations. Without traceability, clinicians and patients are left to trust outputs that may be based on flawed or incomplete data. Furthermore, the lack of reproducibility—the ability to achieve consistent results under similar conditions—compounds these concerns. In high-stakes fields like medicine, this opacity is not just a technical shortcoming but a significant clinical risk.
Take the DermaSensor device as an example. In its FDA filing, the algorithm is described as a “proprietary ML-derived classifier algorithm” that analyzes optical spectroscopy data, specifically the spectrum of scattered light intensity vs wavelength.4 However, the basic scientific foundations connecting this analytical modality to histopathologic correlation are not available for peer review, validation, or protocol review. In addition, the datasets and training processes of the ML models, such as neural network, decision tree, and support vector machine, are not disclosed. In the key study of the efficacy of the device, primary care physicians’ diagnostic accuracy was compared with and without the device. The sensitivity for melanoma detection without the device was found to be 68.8% in the control vs 75.4% with the device. Unfortunately, there is no way to parse where the failures or opportunities for improvement were, and the mechanics for evaluating errors were opaque. Despite this opacity, the FDA’s 513(f)(2) De Novo Classification Letter approving the device did specifically delineate the appropriate use scenario as: 1) to assess lesions already suspicious for skin cancer, not as a screening tool and 2) to assist in the decision regarding referral of the patient to a dermatologist.5
A unique challenge posed by point-of-care devices for lesion classification lies in the need for meticulous documentation and clear accountability in decision-making. Ideally, a clinician using an AI-powered image recognition tool should thoroughly document each lesion assessed, along with the corresponding management plan. Consider a scenario where an amelanotic melanoma is misclassified as benign, and no dermatology referral is initiated— who bears the liability? Is it the clinician relying on the tool, the company providing the device, or the creators of the underlying ML models and datasets? These questions will likely be addressed in future medicolegal cases, but regrettably, not without the cost of potential patient harm.
Conclusion
When it comes to safety-critical software influencing clinical decision-making, reproducible, accurate performance is essential. Although regulators have started to recognize that metadata standards and guidance for traceability in decision-making for devices and software as a medical device are necessary, requirements remain broad. Post-market surveillance is one of the few ways physicians and other users can provide feedback in realworld settings for this type of software. With enough reported issues, it can trigger a recall. Unfortunately, this process necessitates patient harm before action is taken. Dermatologists can help protect patients and promote innovation by approaching AI-powered clinical tools with an appropriate critical eye, understanding that regulations for these tools are still catching up to their rapid development. Standardized metrics and benchmarks for evaluating traceability of AI-powered devices are still being developed, and AI-powered clinical devices are not yet supported by the same data foundations of more mature clinical tools. As always, clinicians must shoulder the responsibility of ensuring patient safety when it comes to AI in medicine and ensure patients receive the highest standard of care.
Disclosure: The author is a member of the AAD DataDerm Oversight Committee. He holds stock in and serves as the CEO of Stratum Biosciences, Inc, a biotechnology start-up based out of JLabs@NYC with significant AI/ML assets for developing skin technology. He has served on advisory boards for Castle Biosciences. He has no commercial interest in any product mentioned in the manuscript.
References
- Wong A, Otles E, Donnelly JP, et al. External validation of a widely implemented prioprietary sepsis prediction model in hospitalized patients. JAMA Intern Med. 2021;181(8):1065-1070. doi:10.1001/jamainternmed.2021.2626
- Ratwani RM, Bates DW, Classen DC. Patient safety and artificial intelligence in clinical care. JAMA Health Forum. 2024;5(2):e235514. doi:10.1001/jamahealthforum.2023.5514
- Elmore JG, Barnhill RL, Elder DE, et al. Pathologists’ diagnosis of invasive melanoma and melanocytic proliferations: observer accuracy and reproducibility study. BMJ. 2017;28;357:j2813. doi:10.1136/bmj.j2813
- De Novo classification request for DemaSensor (DEN230008). US Food and Drug Administration. February 2, 2023. Accessed December 10, 2024. https://www.accessdata.fda.gov/cdrh_docs/reviews/DEN230008.pdf
- De Novo classification order for DermaSensor (DEN230008). US Food and Drug Administration. January 12, 2024. Accessed December 10, 2024. https://www.accessdata.fda.gov/cdrh_docs/pdf23/DEN230008.pdf