Cost and Clinical Implications of Utilizing Homologous Recombination Deficiency Status to Guide First-Line Maintenance Therapy Selection in Advanced Ovarian Cancer
Abstract
Questions remain about the cost of homologous recombination deficiency (HRD) testing to guide the prescribing of poly (ADP-ribose) polymerase (PARP) inhibitors as first-line maintenance for advanced ovarian cancer (AOC). This model evaluates the cost of an HRD biomarker-guided (BMG) approach (olaparib for BRCA-mutated; olaparib and bevacizumab for HRD-positive BRCA wildtype; bevacizumab for HRD-negative) vs a non-BMG approach (niraparib for all patients) for 100 patients with newly diagnosed AOC. The model uses a Markov structure with a US payer perspective and assumes comparisons between therapies based on extrapolated clinical trial results, real-world evidence, and published costs. Despite the upfront cost of HRD testing, a BMG approach is projected to reduce total cost of care by $10 521 642 over 5 years ($51 633 928 vs $62 144 570) and increase progression-free survival in the AOC population (1699 vs 1523 months). This produces $26 in savings for every dollar spent on testing. These data suggest using a BMG approach based on HRD test results to prescribe first-line AOC maintenance therapy may reduce overall cost of care and improve clinical and economic outcomes.
Background
Cancer care is a major focus for the US health care system. As novel therapies to treat various cancer types are developed, the number of cancer survivors and people living with cancer has increased dramatically. Between 2010 and 2020, there was a projected increase of approximately 4.3 million (31%) cancer survivors, and as of 2022, there are more than 18 million Americans living with a history of cancer.1,2 This increase is a testament to progress in cancer care, but it raises concerns about the costs of treatment. According to the Agency for Healthcare Research and Quality, cancer is the second most costly condition for the US health care system.3 The cost of oncology care was projected to increase 39% between 2010 and 2020, which can pose challenges for health care systems.1 In addition to other innovations, such as biomarker testing and imaging, there has been an increase in the complexity and pace of drug discovery in oncology, with a yearly average of 14 novel oncology agents and more new indications for existing drugs approved in the last 5 years.4 Many of these agents are therapies targeted to biomarker-defined patient cohorts, which can result in significant increases in diagnostic and treatment costs to payers alongside improved patient outcomes.
Clinical pathways may represent a strong opportunity to help manage the rising costs and complexity of cancer treatment. In a study of patients with non-small cell lung cancer by the Dana-Farber Cancer Institute, pathway implementation that considered efficacy, toxicity, and cost resulted in savings of over $15 013 per patient, with no significant difference in clinical outcomes compared with before the pathway was implemented.5 Similar results have been observed in gynecologic cancers, where pathway implementation has led to 29% to 32% lower costs, with no appreciable decrease in patient satisfaction (survival not measured).6 These results suggest that appropriate pathway management can be an effective approach to mitigating the mounting costs of cancer care.
Traditional cancer care has long been driven by the anatomic location, stage, and histopathological grading of the disease, but biomarkers and tumor genetic mutations have increasingly influenced oncology trials and approvals.7 Homologous recombination deficiency (HRD) is a phenotype that describes the status of a cell with impaired ability to conduct homologous recombination repair of DNA damage. HRD status can be assessed by two different methods: (1) tests that detect mutations in both BRCA genes (BRCAm) and genomic instability, defined as loss of heterozygosity (LOH), telomeric allelic imbalance and large-scale state transitions in HRD-positive BRCA wildtype (BRCAwt) (MyChoice® CDx test; Myriad Genetic Laboratories, Inc, Salt Lake City, UT); or (2) tests that detect LOH (eg, FoundationOne CDx™; Foundation Medicine, Inc, Cambridge, MA).8 HRD-positive test status is a relevant biomarker in advanced ovarian cancer (AOC). About 50% of patients with AOC have HRD-positive tumors.9 All women with BRCAm AOC are HRD-positive by definition, but not all women who have HRD have a BRCAm. HRD status is relevant to clinical pathways because it can help to inform which patients with AOC may derive greatest survival benefit from targeted maintenance therapies following response to first-line surgery and platinum chemotherapy.10
For patients with AOC, first-line treatment usually consists of surgical removal of the tumor followed by platinum chemotherapy. For patients who respond to chemotherapy, oncologists have the opportunity to prescribe first-line maintenance treatments with the goal of delaying cancer recurrence or even increasing cure rates. Currently, three agents are approved for first-line maintenance. Bevacizumab is an antibody directed against vascular endothelial growth factor that is approved regardless of biomarker status.11 Additionally, there are two poly(ADP-ribose) polymerase (PARP) inhibitors approved in this space. Olaparib was approved in 2018 as monotherapy for patients with a BRCAm and in 2020 as combination therapy with bevacizumab for HRD-positive AOC.12 Niraparib was approved in 2020 for all patients regardless of HRD status.13 In the niraparib PRIMA trials (HRD status assessed using MyChoice® CDx test, HRD-positive test was defined as a BRCAm and/or genomic instability), data support the use of HRD status as a predictive biomarker of response.14,15 Results indicate 24.5 vs 11.2 months’ median progression-free survival (PFS) (niraparib vs placebo) in patients with AOC whose tumors tested HRD-positive (HRD-positive subgroup) and 8.4 vs 5.4 months for the HRD-negative subgroup.13-15 In the PAOLA-1 trial, the HRD-positive subgroup (HRD status assessed using MyChoice® CDx test), who were randomly assigned to receive olaparib plus bevacizumab, had an overall survival (OS) of 66% after 5 years10 and median PFS of 37.2 vs 17.7 months with placebo plus bevacizumab.16 In the SOLO1 trial, patients with BRCAm AOC, a subtype of HRD-positive, who were randomly assigned to receive olaparib, had an OS of 67% after 7 years17 and median PFS of 56.0 vs 13.8 months with placebo.18,19 In PAOLA-1, the HRD-positive BRCAwt and BRCAm subgroups benefited most from addition of olaparib to bevacizumab.16 For patients whose tumors tested HRD-negative or were HRD unknown, median PFS was 16.9 and 16.0 months with olaparib plus bevacizumab and placebo plus bevacizumab, respectively.16 Five-year OS with olaparib plus bevacizumab was 26% vs 32% with placebo plus bevacizumab in patients whose tumors tested HRD-negative, and 31% vs 35% in patients who were HRD-negative/unknown.10 These results suggest that HRD status is predictive of PFS and OS benefit from first-line PARP inhibitor maintenance.10,15,16
Current clinical trial evidence supports multiple approaches to first-line PARP inhibitor usage in AOC. Additional research is needed on which maintenance therapy strategy provides the best value while balancing clinical outcomes and cost. A cost-effectiveness analysis using HRD test results to triage the prescribing of first-line maintenance therapy in AOC could potentially inform pathway and policy decisions related to AOC maintenance treatment. The objective of this study was to model the clinical outcomes and costs associated with utilizing an HRD biomarker-guided (BMG) approach to first-line AOC maintenance compared with a non-biomarker-guided (NBMG) approach.
Methods
To model the impact of HRD testing, we created a multi-state parametric Markov model that simulated a cohort of 100 newly diagnosed ovarian cancer patients over a 5-year period following the completion of first-line treatment (Figure 1). The model allows for patients to pass through six health states including first-line maintenance, second-line treatment, second-line maintenance, third-line treatment, fourth-line treatment, and death or discontinuation. Death or discontinuation is considered to be an absorptive state that patients do not leave. The model estimates the cost of first-line treatment and months of first-line PFS as well as the total cost of treatment (following first-line chemotherapy) for up to 5 years.
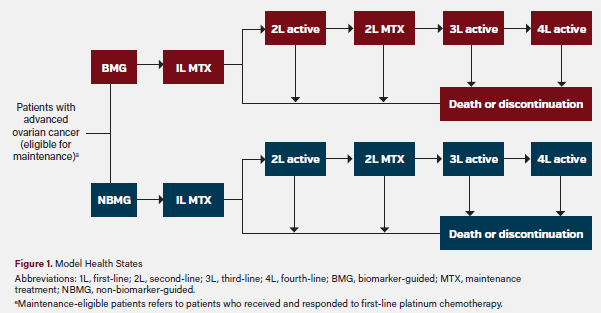
The model compares a BMG treatment scenario with an NBMG treatment scenario. In both scenarios, only patients who received and responded to platinum chemotherapy are eligible for first-line maintenance treatment. In the BMG scenario, HRD test results are used to select a first-line maintenance treatment option. Patients with a BRCAm are assumed to receive olaparib monotherapy. Patients whose tumors tested HRD-positive BRCAwt are assumed to receive olaparib plus bevacizumab, and patients whose tumors tested HRD-negative are assumed to receive bevacizumab monotherapy based on this approach. In the NBMG scenario, all first-line maintenance-eligible patients (who received and responded to platinum chemotherapy) were assumed to receive niraparib. This patient flow is shown in Figure 2.
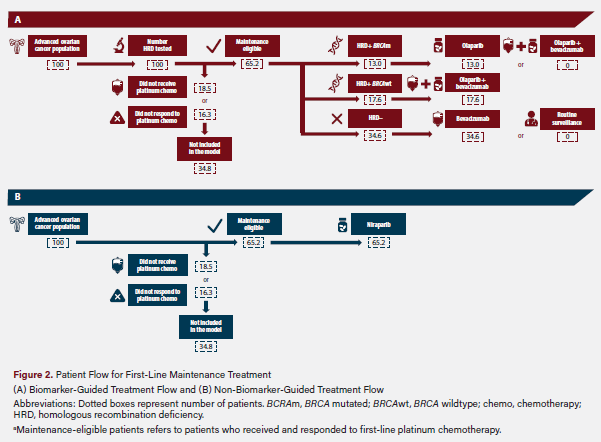
To model first-line PFS for each approach, data from recent, relevant clinical trials were utilized. Kaplan–Meier curves were selected from published trials and digitized using WebPlotDigitizer (version 4.6, 2022, https://automeris.io/ WebPlotDigitizer).13,15,16,18,19 Digitized trial data were import-ed into R, where an algorithm adopted from Guyot et al was applied to obtain patient-level data.20 To extrapolate the survival data, parametric curves were applied to the patient-level data. Exponential, generalized gamma, Gompertz, loglogistic, lognormal, and Weibull curves were tested in R, and the most appropriate curves were selected based on the shape and minimization of Akaike information criterion and Bayesian information criterion (Table 1). Time-dependent hazards were derived from these curves. Generalized Gamma curves were selected to model niraparib, routine surveillance (for sensitivity analysis), and olaparib (BRCAm disease) based on the PRIMA and SOLO1 trials.15,18 A lognormal distribution curve was utilized for the HRD-positive BRCAwt olaparib plus bevacizumab treatment group from the PAOLA-1 trial.16 A loglogistic distribution curve was utilized for the HRD-negative bevacizumab treatment group from the PAOLA-1 trial.16 In the base case, trial data were not adjusted for differences in patient populations.
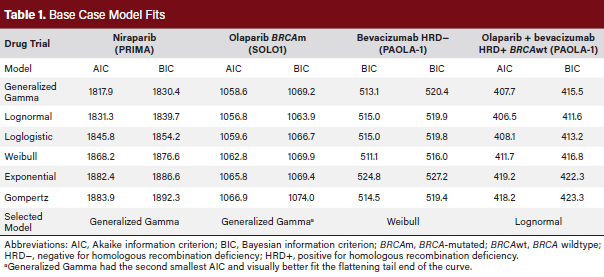
For subsequent lines of therapy, physician surveys have demonstrated a significant degree of variability, with no clear standard of care other than platinum rechallenge for patients meeting criteria for platinum-sensitive disease recurrence.21 For this reason, individualized treatments were not modeled, and the same post-progression treatment was assumed for all arms. Additionally, PFS for each subsequent health state was based on the median value and represented by an exponential curve fit. Second-line active duration was assumed to be 6 months based on physician surveys.21 For the length of second-line maintenance, 14.4 months was used based on a weighted average duration of second-line maintenance therapy for 50% of patients receiving bevacizumab and 50% of patients receiving a PARP inhibitor.22 Third- and fourth-line median duration of therapy was assumed to be 5.5 and 5.0 months, respectively, based on input from expert clinicians. The probability of transitioning between health states was based on data from physician surveys and clinical trials.21,22
The model measures the total cost of care after the end of first-line treatment. Drug acquisition costs, administration costs, and other health care costs were included for first-line maintenance therapies. Drug acquisition costs were based on wholesale acquisition costs and an average monthly number of units. Olaparib dosing was based on the approved US label.12 For niraparib, which utilizes individualized weight- and platelet-based dosing, claims data from Optum’s de-identified Clinformatics® Data Mart Database were evaluated to estimate the average monthly packages. For bevacizumab, which uses weight-based dosing, vials based on the approved package insert, the PAOLA-1 trial protocol, and patient weights from the PAOLA-1 trial were used.16 Based on input from expert pharmacists about real-world practice leading to drug wasting, the number of vials was rounded up if the weight-based dose was higher than 10% of a new vial and rounded down if it was less than 10% of a new vial.
The duration of time on drug was based on trial and label information. Patients receiving olaparib monotherapy who remained progression-free were set to discontinue therapy after 24 months per the SOLO1 and PAOLA-1 trials.16,18 Patients receiving niraparib monotherapy were set to receive treatment until progression per the PRIMA trial and approved label.13,15 For bevacizumab monotherapy and for bevacizumab plus olaparib, the duration of first-line maintenance therapy was set at 11 months from end of chemotherapy based on the PAOLA-1 trial.16
First-line administration costs were based on estimated infusion times for bevacizumab and data from the physician fee schedule.23 First-line adverse event (AE) costs were based on the rate of AEs reported in clinical trials. Grades 3 and 4 AE costs were estimated by multiplying the probability of an event by costs derived from the Healthcare Cost and Utilization Project (HCUP).24 Grade 1 and 2 AEs were assumed to be treated with an outpatient oncology visit, with costing based on the physician fee schedule.24 Due to limited data on the time-dependent nature of AEs, costs were treated as a one-time cost at the start of therapy. Additional monthly health care costs were based on real-world claims data for ovarian cancer patients and include routine visits, pharmacy costs, and other health care expenditures.25 To avoid double counting costs due to maintenance, total health care costs were based on patients who did not receive PARP inhibitor maintenance treatment. All historic costs were adjusted to 2022 costs based on the medical inflation rate, using an inflation calculator (https://www.halfhill.com/inflation_js.html).
HRD testing costs were estimated using the list price for the Myriad MyChoice® CDx HRD test as it is the companion diagnostic for olaparib plus bevacizumab.12,26 For subsequent therapy costs, real-world claims data were utilized to estimate the total subsequent costs.25 Due to the variability in treatment patterns in subsequent lines, claims data may provide a more accurate source of total costs due to the number of assumptions that would be necessary for a drug-specific costing. Monthly costs were assumed to be consistent post-progression regardless of first-line treatment due to limited published data on post-maintenance treatment patterns. A summary of cost inputs can be found in Table 2.11,12,15,16,24,25,26
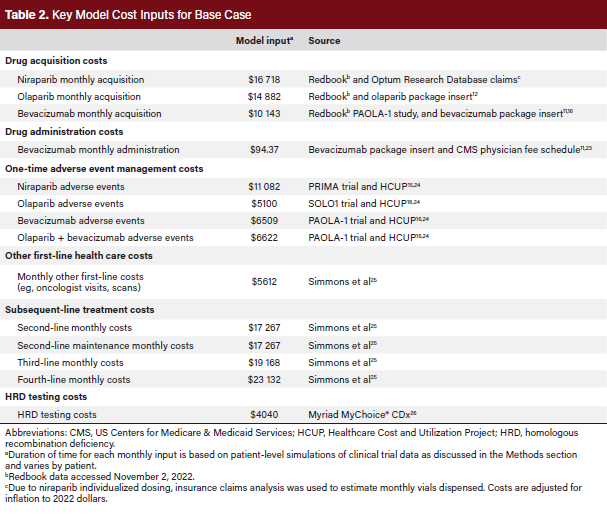
Several deterministic sensitivity analyses were conducted to test the impact of model assumptions on cost per month of PFS. The first scenario tested the impact of 50% of patients with HRD-negative disease receiving routine surveillance in the BMG approach, as opposed to first-line maintenance therapy with bevacizumab. This was modeled because real-world insights suggest that not all of these patients (50% of all AOC) receive maintenance treatments. The second scenario tested the impact of individualized niraparib dosing based on weight and platelet counts. This scenario was modeled to account for the fact that some niraparib patients in the real world may receive lower amounts of drug based on their patient characteristics. The third scenario included the upfront costs of bevacizumab during chemotherapy and as first-line maintenance mono-therapy vs combination therapy with olaparib. This scenario was modeled to account for the fact that many patients begin bevacizumab treatment prior to maintenance. In the fourth sensitivity analysis, a one-time cost for patients who died over the modeled period or discontinued all treatments was estimated.27 In the fifth sensitivity analysis we included a conservative 20% reduction in the cost of bevacizumab to account for potential use of biosimilars. The final scenario tested the impact of an extreme potential increase to niraparib’s trial efficacy to account for differences in the patient populations. An additional 30% reduction in the monthly hazard of progression was assumed based on analysis of outcomes in higher-risk vs lower-risk patients in the PAOLA-1 trial.28
Results
As aforementioned in Figure 2 of the study methods, 35 of 100 patients were assumed ineligible to proceed to first-line maintenance because they had not received or did not respond to platinum therapy. In the NBMG arm, the remaining 65 patients all received niraparib as first-line maintenance following first-line chemotherapy. In the BMG arm, 13 of these 65 patients received olaparib monotherapy (BRCAm), 18 received olaparib plus bevacizumab HRD-positive, BRCAwt), and 35 received bevacizumab monotherapy (HRD-negative; rounded to the nearest whole patient). Over the 5-year duration of the model, the BMG group had 1699 months of first-line PFS compared with 1523 months in the NBMG group.
In addition to producing a higher total number of months of PFS in the population, using a BMG approach yielded a net saving of $10 521 642 compared with using an NBMG approach over the duration of the model ($51 633 928 vs $62 155 570) (Table 3). Cost savings in the BMG approach were largely driven by a reduction in first-line maintenance costs as well as by small reductions in subsequent lines of therapy due to longer first-line PFS. The total cost of first-line therapy was lower for the BMG group than the NBMG group (Table 4). The average cost of first-line maintenance per month of PFS was $14 569 in the BMG model and $22 805 in the NBMG model (Table 4). This translates to the BMG approach saving of $8236 per month of PFS compared with an NBMG approach. A total of $404 000 were spent on HRD testing in the BMG approach yielding a return on investment of $26 saved over the course of the model for every dollar spent on testing.
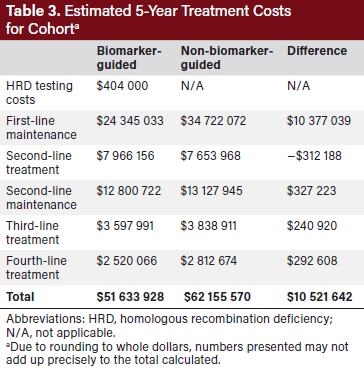
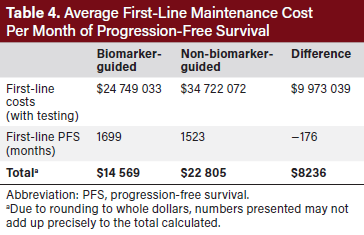
The reduced cost per month of PFS was consistent across all sensitivity analysis scenarios (Table 5). The magnitude of cost savings across the scenarios ranged from $6749 to $8845 per month of PFS, favoring the BMG approach. Total cost savings ranged from $4 988 250 to $15 004 149, favoring the BMG approach.
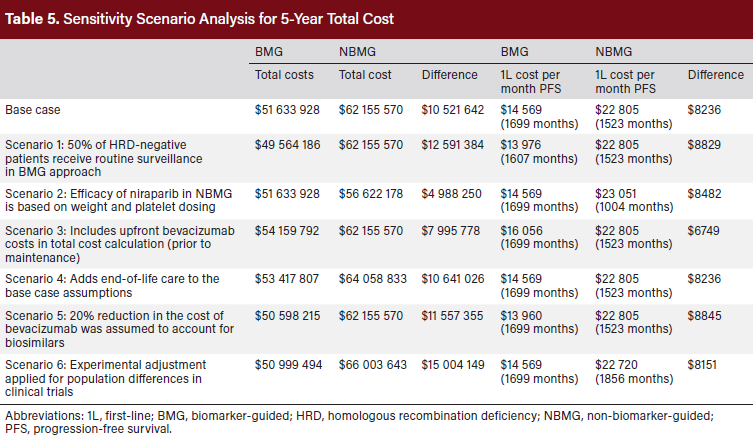
Discussion
This research suggests that using a BMG approach to prescribing first-line maintenance therapy for AOC is projected to improve population clinical outcomes and reduce the total cost of care to the health care system. By utilizing HRD testing in select patients who will benefit most from first-line maintenance with a PARP inhibitor, this model shows an increase in overall months of first-line PFS compared with a population who does not have treatments selected with HRD testing. Using HRD as a predictive biomarker can lead to substantial cost savings for the health care system. Although HRD testing has an upfront cost, it can be viewed as an investment with a high potential clinical and financial return and should be strongly considered for inclusion in clinical pathways.
It is important to consider the value of biomarker testing in the context of rising health care costs. Precision medicine is increasing in importance as we advance molecular profiling of cancer and has the potential to make a positive financial impact on the health care system while improving population health.29 This research adds to data from other tumor types in highlighting the value of emerging biomarkers in oncology. For example, in colorectal cancer, a recent systemic literature search demonstrated that biomarker testing was mostly cost effective or improved the cost effectiveness of targeted therapies.30 In a study by Banerjee et al, insurance coverage was reported to be the number one reason for the lack of biomarker testing (BRCA and HRD) in AOC.31
The results of this model add to the body of evidence in oncology supporting the value of using a BMG approach to prescribing maintenance therapy in AOC. A recent study showed that the cost of treating AOC increases substantially post-progression, underscoring the benefit of prescribing first-line maintenance therapy to either delay or prevent recurrence in as many patients as possible.25 When selecting maintenance, recent publications have demonstrated that maintenance therapy in the BRCA and HRD-guided subgroups are cost effective. For BRCAm patients, olaparib monotherapy has a 99% probability of being cost effective at a willingness-to-pay of $100 000/quality-adjusted life-year (QALY) and generates 2.93 additional QALYs ($51 986/QALY).32 For patients whose tumors tested HRD-positive, combination olaparib plus bevacizumab has a 97% probability of being cost effective at a willingness-to-pay of $100 000/QALY and generates 2.89 QALYs ($56 863/QALY).33 Niraparib was also found to be cost effective for patients whose tumors tested HRD-positive, demonstrating an incremental cost-effectiveness ratio of $56 329/QALY.34 However, this increases substantially to $88 741/QALY in patients whose tumors tested HRD-negative, suggesting a lower probability of being cost effective at a willingness-to-pay threshold of $100 000.34 This study continues to highlight that HRD testing delivers strong value to payers and pathways by helping to select patients for whom PARP inhibitors are predicted to deliver the largest clinical benefit and to be the most cost effective.
One of the strengths of this model is that it considers the cost of treating AOC to the health care system, including drug acquisition costs, administration costs, AE management, diagnostic costs, and other health care expenditure. Furthermore, inputs for cost are based on real-world evidence in order to prioritize their relevance to payers and the health care system. The model is limited by the uncertainty of long-term outcomes for the therapies. Because of their recent approvals, most treatments in the model do not have long-term real-world outcomes data for all regimens in the model. To address this, the model used extrapolation techniques based on clinical trial results. While commonly accepted methodology was utilized, it cannot account for all real-world uncertainty in outcomes. An example of this uncertainty is that the clinical trials selected patients with different baseline characteristics. Sensitivity analyses were performed to attempt to adjust for these differences based on the results of indirect treatment comparisons and clinician insights, but some uncertainty remains. Additionally, this research focuses on PFS instead of OS as a key clinical endpoint due to the immaturity of OS data in this population at the time the model was developed. Because treatment patterns are so diverse after first-line maintenance, the same monthly costs were assumed after first-line maintenance. In the real world, there may be opportunity for cost savings by decreasing variability in this space. Drug costs were taken from list prices in Redbook, and the Optum Research Database was used for evaluating average monthly packages. Data from patients in the Clinformatics® dataset may not represent all health insurance networks or single payer systems. Although in our final sensitivity analysis we reduced the cost of bevacizumab by 20%, there will truly be more cost savings observed as biosimilar utilization increases over time. A study conducted by the RAND Corporation investigating potential cost savings with biosimilars in the US estimated 30% uptake of biosimilars replacing biologics and about a 30% reduction in cost before rebates.35 Hence, the BMG approach would demonstrate lower cost and more savings would be observed since bevacizumab is utilized in both the HRD-positive BRCAwt and the HRD-negative groups. Future studies to further support clinical pathway development by modeling post-progression cost and survival outcomes could be highly useful. Lastly, our model also only captures 5 years of follow-up, the current median OS for AOC. There will always be a need for iterative research with updated modeling as patients live longer with new and improved treatment options.
Conclusion
This model suggests that using an HRD BMG approach to prescribing first-line maintenance in AOC may improve overall population PFS and substantially reduce costs to the health care system compared with an NBMG approach. The model suggests a substantial return on investment for HRD testing, and it should be considered a part of the clinical pathways.
Author Information
Affiliations:
1Oncology Outcomes Research, AstraZeneca Pharmaceuticals LP, Gaithersburg, MD; 2Kelly Gynecologic Oncology Service, Johns Hopkins University School of Medicine, Baltimore, MD; 3Oncology Health Economics & Payer Analytics, AstraZeneca Pharmaceuticals LP, Cambridge, UK; 4US Payer Medical, AstraZeneca Pharmaceuticals LP, Gaithersburg, MD
Funding:
This study was supported by AstraZeneca and Merck Sharp & Dohme LLC, a subsidiary of Merck & Co, Inc, Rahway, NJ, who are codeveloping olaparib.
Correspondence:
Daniel Simmons, PharmD, MS
Oncology Outcomes Research
AstraZeneca Pharmaceuticals LP
1 Medimmune Way, Gaithersburg, MD 20878
Phone: +1 973-970-0364
Email: daniel.simmons@astrazeneca.com
Acknowledgments:
Medical writing assistance was provided by Ben Drever, PhD, of Comradis, and was funded by AstraZeneca and Merck Sharp & Dohme LLC, a subsidiary of Merck & Co, Inc, Rahway, NJ. We thank Zulikhat Segunmaru (AstraZeneca Pharmaceuticals LP) for editorial support and Julia Engstrom-Melnyk (formerly of AstraZeneca Pharmaceuticals LP) for scientific support.
Disclosures:
D.S., J.B., J.R., and K.M. are employees of AstraZeneca. R.S. received research funding from AstraZeneca.
References
1. Mariotto AB, Yabroff KR, Shao Y, Feuer EJ, Brown ML. Projections of the cost of cancer care in the United States: 2010-2020. J Natl Cancer Inst. 2011;103(2):117-128. doi:10.1093/jnci/djq495
2. Miller KD, Nogueira L, Devasia T, et al. Cancer treatment and survivorship statistics, 2022. CA Cancer J Clin. 2022;72(5):409-436. doi:10.3322/caac.21731
3. Soni A. Top five most costly conditions among adults age 18 and older, 2012: estimates for the U.S. civilian noninstitutionalized population. Statistical Brief (Medical Expenditure Panel Survey (US). Agency for Healthcare Research and Quality; 2001.
4. US Food and Drug Administration. Novel drug approvals for 2017–2021. Accessed December 2022. https://www.fda.gov/drugs/development-approval-process-drugs/new-drugs-fda-cders-new-molecular-entities-and-new-therapeutic-biological-products
5. Jackman DM, Zhang Y, Dalby C, et al. Cost and survival analysis before and after implementation of Dana-Farber clinical pathways for patients with stage IV non-small-cell lung cancer. J Oncol Pract. 2017;13(4):e346-e352. doi:10.1200/jop.2017.021741
6. Ghosh K, Downs LS, Padilla LA, et al. The implementation of critical pathways in gynecologic oncology in a managed care setting: a cost analysis. Gynecol Oncol. 2001;83(2):378-382. doi:10.1006/gyno.2001.6428
7. Renfro LA, An MW, Mandrekar SJ. Precision oncology: a new era of cancer clinical trials. Cancer Lett. 2017;387:121-126. doi:10.1016/j.canlet.2016.03.015
8. Konstantinopoulos PA, Ceccaldi R, Shapiro GI, D’Andrea AD. Homologous recombination deficiency: exploiting the fundamental vulnerability of ovarian cancer. Cancer Discov. 2015;5(11):1137-1154. doi:10.1158/2159-8290.CD-15-0714
9. Integrated genomic analyses of ovarian carcinoma. Nature. 2011;474(7353):609-615. doi:10.1038/nature10166
10. Ray-Coquard I, Leary A, Pignata S, et al. Olaparib plus bevacizumab first-line maintenance in ovarian cancer: final overall survival results from the PAOLA-1/ENGOT-ov25 trial. Ann Oncol. 2023;34(8):681-692. doi:10.1016/j.annonc.2023.05.005
11. Avastin. Prescribing Information. Genetech; 2022.
12. Lynparza. Prescribing information. Astrazeneca; 2023.
13. Zejula. Prescribing information. GlaxoSmithKline; 2024.
14. Gonzalez Martin AJ, Pothuri B, Vergote IB, et al. 530P PRIMA/ENGOT-OV26/GOG- 3012 study: updated long-term PFS and safety. Ann Oncol. 2022;33(suppl 7):S789. doi:10.1016/j.annonc.2022.07.658
15. González-Martín A, Pothuri B, Vergote I, et al. Niraparib in patients with newly diagnosed advanced ovarian cancer. N Engl J Med. 2019;381(25):2391-2402. doi:10.1056/NEJMoa1910962
16. Ray-Coquard I, Pautier P, Pignata S, et al. Olaparib plus bevacizumab as first-line maintenance in ovarian cancer. N Engl J Med. 2019;381(25):2416-2428. doi:10.1056/ NEJMoa1911361
17. DiSilvestro P, Banerjee S, Colombo N, et al. Overall survival with maintenance olaparib at a 7-year follow-up in patients with newly diagnosed advanced ovarian cancer and a BRCA mutation: the SOLO1/GOG 3004 trial. J Clin Oncol. 2023;41(3):609- 617. doi:10.1200/JCO.22.01549
18. Moore K, Colombo N, Scambia G, et al. Maintenance olaparib in patients with newly diagnosed advanced ovarian cancer. N Engl J Med. 2018;379(26):2495-2505. doi:10.1056/NEJMoa1810858
19. Banerjee S, Moore KN, Colombo N, et al. Maintenance olaparib for patients with newly diagnosed advanced ovarian cancer and a BRCA mutation (SOLO1/GOG 3004): 5-year follow-up of a randomised, double-blind, placebo-controlled, phase 3 trial. Lancet Oncol. 2021;22(12):1721-1731. doi:10.1016/s1470-2045(21)00531-3
20. Guyot P, Ades AE, Ouwens MJ, Welton NJ. Enhanced secondary analysis of survival data: reconstructing the data from published Kaplan-Meier survival curves. BMC Med Res Methodol. 2012;12:9. doi:10.1186/1471-2288-12-9
21. CernerEnviza. Treatment Architecture: United States Ovarian Cancer. CancerMPact; 2018.
22. Pujade-Lauraine E, Ledermann JA, Selle F, et al. Olaparib tablets as maintenance therapy in patients with platinum-sensitive, relapsed ovarian cancer and a BRCA1/2 mutation (SOLO2/ENGOT-Ov21): a double-blind, randomised, placebo-controlled, phase 3 trial. Lancet Oncol. 2017;18(9):1274-1284. doi:10.1016/s1470-2045(17)30469-2
23. Physician fee schedule. US Centers for Medicare & Medicaid Services. Accessed October 13, 2024. https://www.cms.gov/medicare/payment/fee-schedules/physician
24. Healthcare Cost and Utilization Project. Rockville, MD: Agency for Healthcare Research and Quality; 2022.
25. Simmons D, Blank SV, ElNaggar AC, Chastek B, Bunner SH, McLaurin K. Health care resource utilization and costs associated with disease progression in ovarian cancer. Adv Ther. 2022;39(6):2544-2561. doi:10.1007/s12325-022-02086-5
26. Advanced diagnostic laboratory tests under the Medicare CLFS. US Centers for Medicare & Medicaid Services. Accessed November 2, 2022. https://www. cms.gov/Medicare/Medicare-Fee-for-Service-Payment/ClinicalLabFeeSched/ Downloads/List-of-Approved-ADLTs.pdf
27. Poonawalla IB, Parikh RC, Du XL, VonVille HM, Lairson DR. Cost effectiveness of chemotherapeutic agents and targeted biologics in ovarian cancer: a systematic review. Pharmacoeconomics. 2015;33(11):1155-1185. doi:10.1007/s40273-015-0304-9
28. Harter P, Mouret-Reynier MA, Pignata S, et al. Efficacy of maintenance olaparib plus bevacizumab according to clinical risk in patients with newly diagnosed, advanced ovarian cancer in the phase III PAOLA-1/ENGOT-ov25 trial. Gynecol Oncol. 2022;164(2):254-264. doi:10.1016/j.ygyno.2021.12.016
29. Ginsburg GS, Phillips KA. Precision medicine: from science to value. Health Aff (Millwood). 2018;37(5):694-701. doi:10.1377/hlthaff.2017.1624
30. Seo MK, Cairns J. Do cancer biomarkers make targeted therapies cost-effective? A systematic review in metastatic colorectal cancer. PLoS One. 2018;13(9):e0204496. doi:10.1371/journal.pone.0204496
31. Banerjee S, Valabrega G, Bailey T, et al. Physician behavior and perceptions around genetic biomarker test utilization for the management of newly diagnosed advanced ovarian cancer patients: US physician survey. Gynecol Oncol. 2022;166:S124. doi:10.1016/S0090-8258(22)01448-2
32. Muston D, Hettle R, Monberg M, et al. Cost-effectiveness of olaparib as a maintenance treatment for women with newly diagnosed advanced ovarian cancer and BRCA1/2 mutations in the United States. Gynecol Oncol. 2020;159(2):491-497. doi:10.1016/j.ygyno.2020.08.013
33. Elsea D, Fan L, Mihai A, et al. Cost-effectiveness analysis of olaparib in combination with bevacizumab compared with bevacizumab monotherapy for the first-line maintenance treatment of homologous recombination deficiency-positive advanced ovarian cancer. Pharmacoecon Open. 2022;6(6):811-822. doi:10.1007/s41669-022-00338-2
34. Barrington DA, Tubbs C, Smith HJ, Straughn JM, Jr, Senter L, Cohn DE. Niraparib maintenance in frontline management of ovarian cancer: a cost effectiveness analysis. Int J Gynecol Cancer. 2020;30(10):1569-1575. doi:10.1136/ijgc-2020-001550
35. Mulcahy AW, Hlavka JP, Case SR. Biosimilar cost savings in the United States: initial experience and future potential. Rand Health Q. 2018;7(4):3.