Artificial Intelligence in Oncology: The Wins, The Challenges, and How We Can Deliver on Personalized Cancer Care
Abstract
The development of effective, targeted therapies for cancer remains one of the primary goals in precision medicine, requiring the input of multiple disciplines across the diagnostic and therapeutic landscape. Despite the significant role of cancer care in medicine, there is a forecasted shortfall of physicians able to diagnose and treat patients along their cancer diagnosis journey, which is only projected to worsen in the coming decades. Artificial intelligence (AI), and its manifestations in both machine learning and deep learning, are poised to unburden physicians from many laborious and repetitive tasks in diagnostic analyses, while also providing insight into prospective therapeutic options. AI will increasingly facilitate decision-making as well as improve the accuracy and relevance of therapeutic recommendations. This collaborative interaction between algorithms and physicians promises to enhance the field of precision medicine and further enable optimal patient care.
The Precision Oncology Landscape
Recent technological and computational advances have transformed how we address ambiguity in medicine, offering a glimpse into a future with new methods for predicting patient outcomes based on the analysis of massive data sets. Developments in analyzing these “big data” repositories present an opportunity, particularly in cancer care, where outcome prediction and optimal therapy choice are critical to successful treatment. With the development of advanced artificial intelligence (AI), machine-learning algorithms, and enhanced analytical models, predictive technologies are poised to present more accurate prognoses of cancer and identify the best therapeutic options for individual patients.1,2 At SimBioSys, our aim is to advance these crucial patient care goals through a combination of patient-specific medical imaging, AI-enabled analysis, and metabolic modeling, which together comprise a new dimension in personalized cancer care.3-5
The US Food and Drug Administration (FDA) describes precision medicine as tailoring “disease prevention and treatment for individual variability (eg, genetic and lifestyle differences among patients)” with the goal to “match the right treatments for each individual patient at the right time.”6 In the context of cancer care, precise medicine options represent an end-to-end treatment journey encompassing a wide range of modalities, including risk stratification, precision screening, precision diagnostics, precision tumor profiling, precision treatment, precision dosing, and precision monitoring. These areas must be unified to have substantive and groundbreaking impact. Here, we described precision medicine specifically as it relates to customizing and optimizing therapies in the context of cancer care.
The Wins
Precision oncology encompasses the array of technologies and therapies that enable the targeted treatment of cancer based on the disease’s genetic, transcriptional, or molecular profile. The field aims to elaborate the mechanistic understanding of cancer development and progression and use these insights as the foundation for diagnostics and therapeutics that are targeted to an individual’s unique background and medical history. Consideration of a patient’s overall health, age, and preexisting conditions are all crucial factors to ensure that their treatment plan is optimized to meet their unique needs. However, current medical guidelines are broadly based on population-level statistics, rather than individually tailored treatment planning. These large-scale data sets can be used to stratify individual risk and inform screening strategies to triage patients and customize their treatment plans. This strategy still lags behind ambitions to truly tailor therapies on an individual level.
There is a clear demand from patients to better understand medical diagnoses, as reflected in their overwhelming desire to have early access to medical imaging and cancer diagnostic procedure data.7,8 Personalized medicine offers numerous opportunities for enhanced individualized treatment selection and optimization throughout a patient’s life (Figure 1). Genomics now routinely provides valuable insights into tumor behavior, but this information must be considered alongside additional risk factors and patient characteristics. For example, a patient’s lifestyle and environment can significantly influence their risk of developing cancer and the effectiveness of treatment—aspects that are difficult to capture with laborious human review of patient records, but which are well-suited for analysis by automated data-mining methods. The integration of patient-specific data into treatment planning will undoubtedly benefit from the analytical efficiency and multiplexed capabilities of AI.9
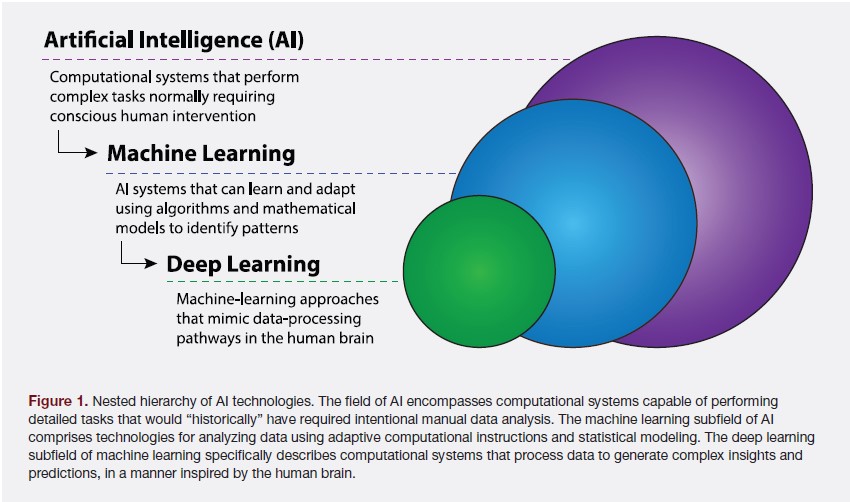
The Challenges
The need for cancer treatment is expected to grow significantly in the coming years, with a 40% projected increase in demand.10 At the same time, the American Society of Clinical Oncology has projected a shortage of more than 2200 oncologists in the US by 2025.10 Although new mechanisms to funnel additional health care workers into the field have been proposed,11 even a robust combination of strategies cannot meet the ever-expanding demand using human labor alone. This suggests that AI and other technological tools will likely play an increasingly important role in cancer care, helping automate routine tasks and allowing oncologists to focus on more complex cases and personalized treatment plans. Ultimately, a combination of human expertise and technological tools will be necessary to provide high-quality and personalized cancer care to all patients in need.
Since 1995, the FDA has approved over 500 AI-enabled applications in medicine, of which 75% have occurred within medical imaging.12,13 However, there remains significant potential for AI expansion into fields beyond radiology. AI has already demonstrated promise in various health care domains, such as drug development, genomics, and clinical decision-making. For instance, AI can be used to analyze large volumes of genomic data to identify potential drug targets or develop personalized treatment plans for patients. Additionally, AI can help clinicians make more accurate diagnoses by processing and analyzing data from various sources, such as electronic health records, medical images, and laboratory tests.
Machine-learning approaches have shown great promise in streamlining radiologic information extraction and analysis, enabling data from magnetic resonance imaging scans to build 3-dimensional representations automatically. This not only helps radiologists spend less time on repetitive tasks but also allows them to focus more on interacting with other physicians or patients. In a survey of approximately 3000 radiologists, AI was rated as having the most significant impact on breast, neuro-, oncologic, and thoracic imaging. Notably, 55% of survey respondents indicated that AI-only reports would not be acceptable to patients.14 A parallel result by Pew Research indicates that 60% of patients self-report that they would be uncomfortable with their provider relying on AI for directing their treatment.15
As with any new technology, AI approaches in medicine face imminent challenges to ensure their accuracy and reliability. Models and prognostic recommendations are only as good as the data on which they are trained.16 Hence, as new therapy response data sets are made available, the relevant recommendations will shift to better reflect these state-of-the-art developments; and although AI can help identify the optimal treatment regimen for a given patient, it requires continuous updating and retraining to remain accurate.16 AI enables informed decision-making that ultimately benefits patients, but it is critical that these processes align with ethical principles and morality. For instance, AI has highlighted biases in health care due to human language usage and historical social structures,17 which require conscious effort to rectify.18,19
Despite these obstacles, AI already has successful applications in analyzing medical imaging, both from a diagnostic and outcome-prediction perspective. The fields of pulmonology and cardiology have numerous FDA-approved AI implementations, particularly for predicting sites of blood flow restriction, perfusion impairment, and clot formation.20
Machine-learning methodologies have clear advantages and efficiencies over laborious manual data curation.21 Advances in natural language processing enable machines to contextualize language and assemble complex connections between words (ideas) and the data they describe. The recent advent of generative AI utilizes computational “neural networks” operating in a manner that is conceptually parallel to the human brain. The fluidity and adaptability of generative AI, when fully implemented across the health care landscape, will be crucial for extracting the trove of medical information that lies within the estimated 80% of medical data that is unstructured and thus inaccessible to current computational data-analysis tools.22
Generative AI algorithms process and integrate the array of existing observational patient data to produce a recommended treatment plan.23 The ability to integrate multiscale data sets into the patient treatment pipeline is enabled by recent advances in “transformers,” which power ever-larger models without having to manually label the data in advance. In addition, transformers have unlocked a new notion called “attention” that enables models to track features across images as they evolve over time in response to treatment. These properties of generative AI are primed for applications within the medical landscape,24 so long as the questions asked fall within a spectrum of known or closely related medical information.25
Delivering on the Promise of Personalized Cancer Care Through Guided Intelligence
Early implementations of generative AI in medicine have been plagued by so-called “hallucinations” that output inaccurate, sometimes illogical, prognoses and recommendations, often presented with alarming degrees of confidence.26 These problematic diagnoses often reflect the necessity of further refinements to the underlying AI model, but also may be founded in inherent flaws in the underlying “ground truth” data.27 Generative AI cannot currently appreciate, nor compensate for, a lack of curated structure or incomplete, erroneous, or biased data sets. Before AI systems can integrate these records into informed medical decision-making, additional layers of data curation will be needed to assure semantic and data-structure interoperability. The OpenAI Codex initiative facilitates the translation of natural language in medical records into structured code,28 and further developments in this area are crucial for expanding the breadth and accuracy of the indexed data sets, as well as counterbalancing (rather than echoing) the human biases contained therein.
Computational tools are expressly not intended to replace, nor do they have the capability to replace, the expertise of physicians. The goal of AI at the cutting edge of medicine is guided intelligence, incorporating elements of AI and mathematical modeling to support and empower physicians in their decision-making process. AI can serve to augment and enhance clinical decision-making, rather than replace human judgment and expertise. Individual physicians can have vastly different treatment recommendations, such that a single patient could receive a wide range of possible therapies based on their physician’s history and experiences. The future of AI is in identifying which individual is best suited for these treatment possibilities.
Taken together, these advances demonstrate that future medical applications of AI will place these technologies not as decision-makers, but as decision-supporters. AI-enabled analyses will become crucial tools to help identify treatment options for each individual patient alongside a forecasted outcome (Figure 2). AI is primed to enable more collaborative treatment decision-making as well as focus therapeutic recommendations (ie, highlighting the optimal treatment at the optimal time).
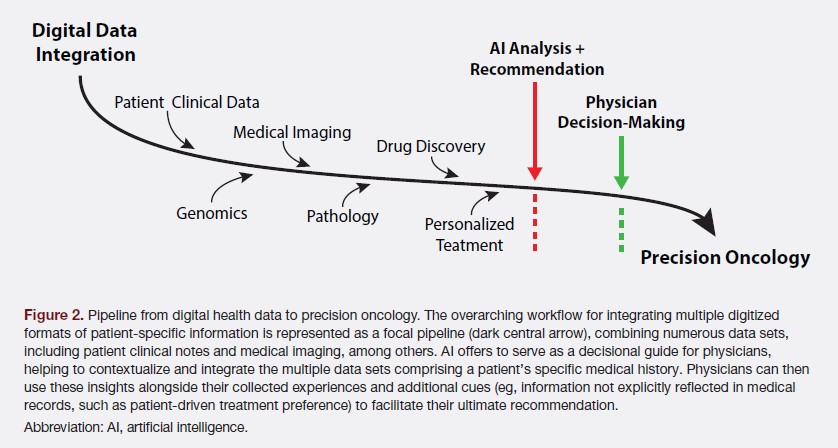
We envision that the time saved by AI in the cancer care pipeline can be used to foster stronger interaction between radiologists, clinicians, and patients. By enabling more personal interactions, medical oncologists can delve deeper into patient-specific treatment needs and provide better overall care. Time allocation will therefore shift to enable physicians to dive deeper into their patients’ treatments and ultimately drive better therapeutic outcomes.
New technologies will streamline workflows and help address the increasing time constraints on physicians, and AI integration into the oncology pipeline will ultimately provide better outcomes for patients. AI is already capable of managing the low-hanging fruit of the physician workload automatically—and as these abilities continue to advance in sophistication, AI will increasingly free physicians to dedicate their attention to the nuances of patient care. AI will enable even greater degrees of personalization and usher in a new era in identifying and developing therapeutic options customized to each individual.
AI is here to stay, and rather than resist it, we argue for a new implementation of AI that combines machine intelligence with the guidance and critical thinking of human experts. AI’s ability to predict treatment responses and simulate surgical outcomes will undoubtedly enhance physicians’ treatment toolkits. Our perspective is that patients and their physicians will remain the ultimate decision-makers in the patient care pipeline, where they will exercise their combined perspectives and insights to balance between efficacy, toxicity, cost, and benefit of different treatment options. Insights from AI will undoubtedly revolutionize health care, but it is essential to understand its limitations relative to the expertise of the physicians who can assess the utility and applicability of these insights for a particular patient.
Cancer caregivers can therefore embrace AI for its utility within a specific scope. Take heart that medicine will not be overrun by intelligent machines; the fundamentally human element of the patient-physician dynamic cannot be replaced.
References
1. Iqbal MJ, Javed Z, Sadia H, et al. Clinical applications of artificial intelligence and machine learning in cancer diagnosis: looking into the future. Cancer Cell Int. 2021;21:270. doi:10.1186/s12935-021-01981-1
2. Chen MM, Terzic A, Becker AS, et al. Artificial intelligence in oncologic imaging. Eur J Radiol Open. 2022;9:100441. doi:10.1016/j.ejro.2022.100441
3. Howard FM, He G, Peterson JR, et al. Highly accurate response prediction in high-risk early breast cancer patients using a biophysical simulation platform. Breast Cancer Res Treat. 2022;196(1):57-66. doi:10.1007/s10549-022-06722-0
4. Cook D, Biancalana M, Liadis N, et al. Next generation immuno-oncology tumor profiling using a rapid, non-invasive, computational biophysics biomarker in early-stage breast cancer. Front Artif Intell. 2023;6. doi:10.3389/frai.2023.1153083
5. Peterson JR, Cole JA, Pfeiffer JR, et al. Novel computational biology modeling system can accurately forecast response to neoadjuvant therapy in early breast cancer. Breast Cancer Res. 2023;25(1):54. doi:10.1186/s13058-023-01654-z
6. FDA. Focus Area: Individualized Therapeutics and Precision Medicine. Last reviewed September 6, 2022. Accessed June 1, 2023. https://www.fda.gov/science-research/focus-areas-regulatory-science-report/focus-area-individualized-therapeutics-and-precision-medicine
7. Steitz BD, Turer RW, Lin CT, et al. Perspectives of patients about immediate access to test results through an online patient portal. JAMA Netw Open. 2023;6(3):e233572. doi:10.1001/jamanetworkopen.2023.3572.
8. PocketHealth Inc. Patients Speak Out On the State of Healthcare: New Patient Pulse survey finds patients eager to play a larger role in their care, with those who do citing a better healthcare experience. Cision PR Newswire. July 21, 2022. https://www.prnewswire.com/news-releases/patients-speak-out-on-the-state-of-healthcare-new-patient-pulse-survey-finds-patients-eager-to-play-a-larger-role-in-their-care-with-those-who-do-citing-a-better-healthcare-experience-301589944. html
9. Jaber N. Can Artificial Intelligence Help See Cancer in New, and Better, Ways? National Cancer Institute. March 22, 2022. https://www.cancer.gov/news-events/ cancer-currents-blog/2022/artificial-intelligence-cancer-imaging
10. Wenya Yang B, Williams JH, Hogan PF, et al. Projected supply of and demand for oncologists and radiation oncologists through 2025: An aging, better-insured population will result in shortage. J Oncol Pract. 2014;10(1):39-45. doi:10.1200/ JOP.2013.001319
11. Vose JM. The Future of Oncology: Supply and Demand for Oncology Services. Oncology. 2021;35(6). https://www.cancernetwork.com/view/the-future-oncology-supply-demand-for-oncology-services
12. Colangelo M. FDA Publishes Updated List Of 521 Authorized AI Enabled Medical Devices. October 7, 2022. https://www.linkedin.com/pulse/fda-publishes-updated-list-521-authorized-aiml-margaretta-colangelo/
13. Fornell D. FDA has now cleared more than 500 healthcare AI algorithms. HealthExec. February 6, 2023. Accessed July 19, 2023. https://healthexec.com/topics/ artificial-intelligence/fda-has-now-cleared-more-500-healthcare-ai-algorithms
14. Codari M, Melazzini L, Morozov SP, van Kuijk CC, Sconfienza LM, Sardanelli F. Impact of artificial intelligence on radiology: a EuroAIM survey among members of the European Society of Radiology. Insights Imaging. 2019;10(1):105. doi:10.1186/ s13244-019-0798-3
15. Tyson A, Pasquini G, Spencer A, Funk C. 60% of Americans Would Be Uncomfortable With Provider Relying on AI in Their Own Health Care. Pew Research Center. February 22, 2023. https://www.pewresearch.org/science/2023/02/22/60-of-americans-would-be-uncomfortable-with-provider-relying-on-ai-in-their-own-health-care/
16. Rajpurkar P, Lungren MP. The Current and Future State of AI Interpretation of Medical Images. Drazen JM, Kohane IS, Leong T-Y, editors. N Engl J Med. 2023;388(21):1981–1990. doi:10.1056/NEJMra2301725
17. Gichoya JW, Banerjee I, Bhimireddy AR, et al. AI recognition of patient race in medical imaging: a modelling study. Lancet Digit Health. 2022;4(6):e406-e414. doi:10.1016/S2589-7500(22)00063-2
18. Chen IY, Pierson E, Rose S, Joshi S, Ferryman K, Ghassemi M. Ethical machine learning in healthcare: annual review of biomedical data science. Annu Rev Biomed Data Sci. 2021;4:123-144. doi:10.1146/annurev-biodatasci-092820
19. Haug CJ, Drazen JM. Artificial Intelligence and Machine Learning in Clinical Medicine. N Engl J Med. 2023;388(13):1201-1208. doi:10.1056/NEJMra2302038
20. Milam ME, Koo CW. The current status and future of FDA-approved artificial intelligence tools in chest radiology in the United States. Clin Radiol. 2023;78(2):115-122. doi:10.1016/j.crad.2022.08.135
21. Bi WL, Hosny A, Schabath MB, et al. Artificial intelligence in cancer imaging: Clinical challenges and applications. CA Cancer J Clin. 2019;69(2):127-157. doi:10.3322/ caac.21552
22. Rogers A. The 80% Blind Spot: Are You Ignoring Unstructured Organizational Data? Forbes. January 29, 2019. https://www.forbes.com/sites/forbestechcouncil/ 2019/01/29/the-80-blind-spot-are-you-ignoring-unstructured-organizational-data/
23. McKinsey & Company. What is generative AI? January 19, 2023. Accessed June 12, 2023. https://www.mckinsey.com/featured-insights/mckinsey-explainers/ what-is-generative-ai
24. Drazen JM, Kohane IS, Leong T-Y, et al. Benefits, Limits, and Risks of GPT-4 as an AI Chatbot for Medicine. N Engl J Med. 2023;388(13):1233–1239. doi:10.1056/ NEJMsr2214184
25. Lee, P, Bubeck S. Benefits, Limits, and Risks of GPT-4 as an AI Chatbot for Medicine—To the Editor. N Engl J Med. 2023;388(25):2397-2400. doi:10.1056/ NEJMc2305287
26. Lawton G. What is generative AI? Everything you need to know. Tech Target. Last updated July 2023. Accessed June 20, 2023. https://www.techtarget.com/ searchenterpriseai/definition/generative-AI
27. Craig L, Hashemi-Pour C. How data quality shapes machine learning and AI outcomes. TechTarget. July 14, 2023. Accessed June 20, 2023. https://www. techtarget.com/searchenterpriseai/feature/How-data-quality-shapes-machine-learning-and-AI-outcomes
28. OpenAI. OpenAI Codex. 2023. Accessed July 18, 2023. https://openai.com/blog/ openai-codex