Histologic Screening of Melanoma Using a Deep Learning Model
In this interview, Dr Manuel Valdebran and Dan Zhang discuss using a deep learning model and convolutional networks for the histologic screening of malignant melanoma, melanocytic nevi, and Spitz nevi.
Manuel Valdebran, MD, is an assistant professor in the department of dermatology and dermatologic surgery at the Medical University of South Carolina. Dan Zhang is a PhD in computer science student at Clemson University with an MS in computer science from New York University.
The Dermatologist: Can you explain what convolutional networks are and how you utilize them?
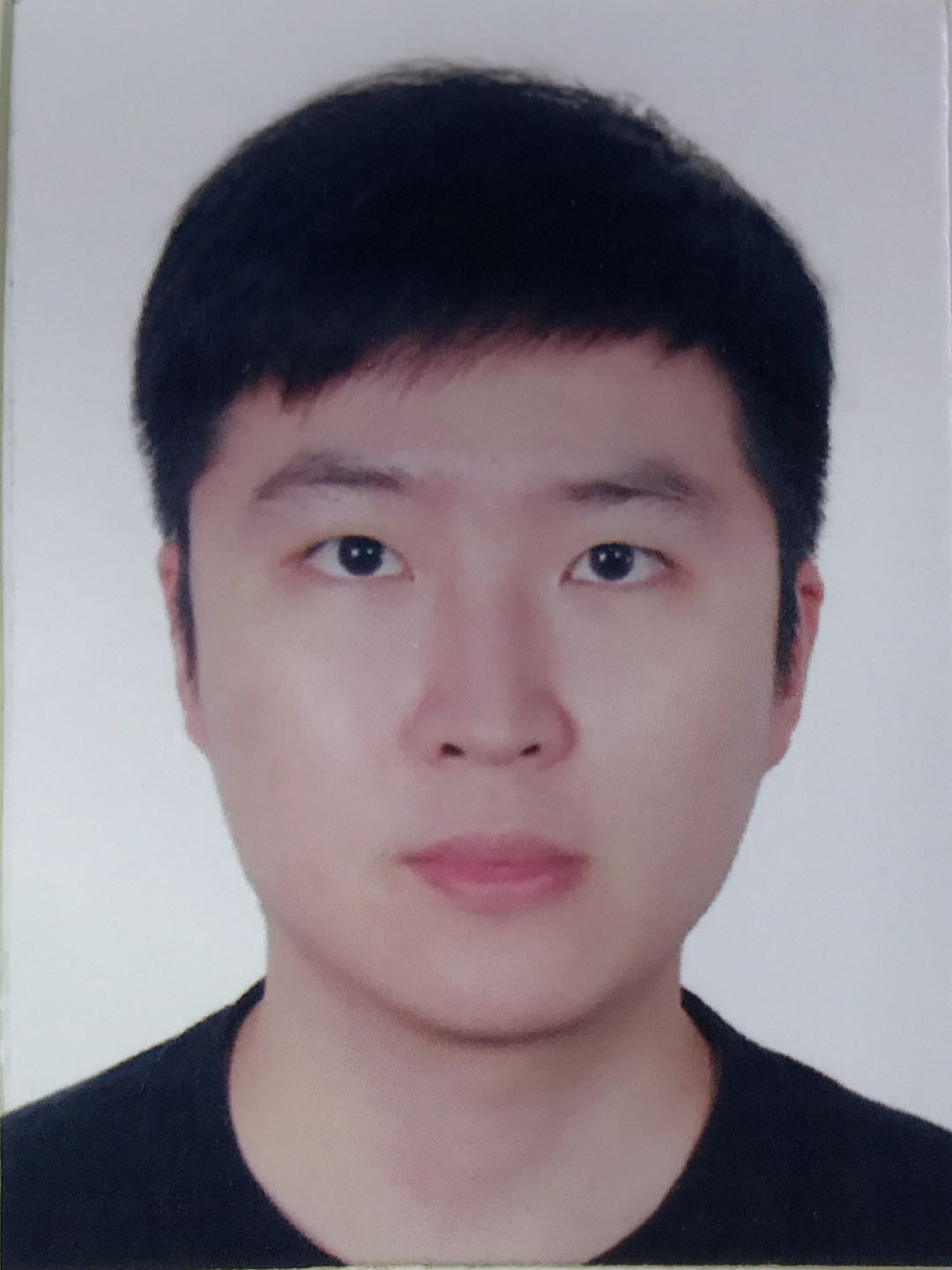
Dan Zhang: The convolutional neural networks, or CNNs, are a type of neural network that is specifically designed for image processing and computer vision tasks. The basic building block of a CNN is the convolutional layer, which applies a set of learnable filters to the input image. And each filter just extracts a specific feature from the image, such as the edges and corners or blobs. After the convolutional layer, there's a pulling layer that down samples the feature map, so you can reduce its size while preserving the most important features from the image.
We also have a fully connected layer as the last layer that performs the final classification task. During the training process, the weights of the filters and the fully connected layers are optimized using the back propagation and creating this algorithm. For a host-like image during the training, we first crop it and train the network using some of the label types. And during the prediction the whole slide image would be labeled as melanoma if most of the tasks from it are classified as melanoma and similar to other categories. That's basically how we train the whole network and how to use it to predict the labels for each image.
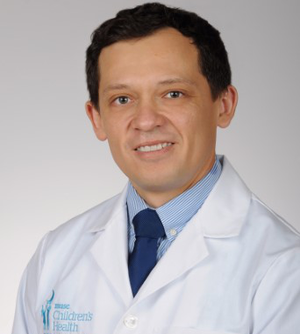
Dr Manuel Valdebran: Convolutional networks are trained in cellular morphology. And this is very interesting because it's kind of a new predicament. It resulted from our experiments from prior studies where we got some data that oriented us to think cytology may be important to differentiate between melanoma and nevi. Based on those experiments, we sought to conceptualize the model and we gave priority to analyzing the cytology as opposed to evaluating the architecture. When we evaluate melanocytic proliferation, we evaluate both architectures and cytology. But since we had this experience before, we gave the cytology a priority. And interestingly, the results of the experiments showed that the model was very robust in terms of predicting melanocytic proliferation based on the cytology. And if you can see on the figures in the hit maps, they demonstrated that cytological and nuclear features and the stroma around these cells are certainly key for the diagnosis of each proliferation.
The Dermatologist: How do you envision the use of algorithms in dermatopathology?
Dan Zhang: We think that algorithms have great potential in dermatopathology and can assist pathologists in making more accurate decisions. From our testing experiments, the images in our testing data set have not been seen by the model during the training process. This means that we can still achieve 85% of the position and the recall for melanoma, demonstrating the robustness and efficiency of diagnoses for skin diseases. And we are working on generalizing our model to the data from other institutions. In this case, we can ensure the consistencies of diagnosis from our model.
Dr Manuel Valdebran: In the final experiment, we got a pretty good sensitivity. We got a 100% sensitivity for detection of melanoma and very good specificity for nevi and Spitz nevi as well. That is what we are requiring from the model so it can serve like a screening tool to help pathologists flag potential cases. In that sense, I think the results of the model are very promising and we hope we can expand the research in this area.
The Dermatologist: What do you think the future of this deep learning model looks like for dermatopathology and dermatology?
Dr Manuel Valdebran: I think that artificial intelligence can help us screen clinical lesions, as well as histological slides, and flag those lesions that are clinically concerning for skin cancer and on the pathological side, flag those cases where they're more likely to be malignant and, ultimately, help patient care. We are envisioning very good things if these models get developed a little bit more.
The Dermatologist: Is there anything else about artificial intelligence you would like to share with your colleagues?
Dr Manuel Valdebran: Artificial intelligence has an exciting potential for detection of features not seen by the human eye. And it can certainly speed up the reading process when talking about pathology and labs processes and can have a very significant impact on patient care both clinically and pathologically. We want to call institutions for collaboration because melanoma is a deadly cancer. If we can get more institutions and get samples to corroborate and create a unified model, it will advance patient care, provide an early and accurate diagnosis, and predict several things like molecular signatures and outcomes as well.
Reference:
Snyder AN, Zhang D, Dreesen SL, et al. Histologic screening of malignant melanoma, Spitz, dermal and junctional melanocytic nevi using a deep learning model. Am J Dermatopathol. 2022;44(9):650-657. doi:10.1097/DAD.0000000000002232