Speech, Language Assessment Useful in Schizophrenia Spectrum Disorders Treatment
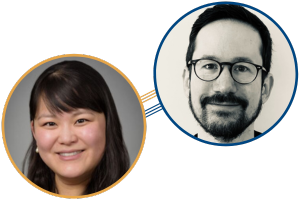
Sunny Tang, MD, and Michael Spilka, PhD, 2023 annual American Psychiatric Association (APA) meeting co-presenters, share how natural language processing (NLP) can be useful in treating schizophrenia, how to integrate NLP into clinical practice, and the limitations of the using these markers. In this Q&A, Dr Tang and Dr Spilka share these highlights and more from their recent session titled, “Diagnosing Schizophrenia in the 21st Century: Natural Language Processing as an Emerging Biomarker."
To stay in the know with the hottest topics from the 2023 Annual Meeting, visit our APA Key Clinical Topics hub.
Meagan Thistle, Psych Congress Network: What were the main takeaways from your 2023 APA session “Diagnosing Schizophrenia in the 21st Century: Natural Language Processing as an Emerging Biomarker”?
Michael Spilka, PhD, and Sunny Tang, MD: Speech provides a rich source of information about ongoing illness processes in schizophrenia, yet our traditional clinical assessments of language disturbance are time-intensive and subjective. Computational and natural language processing (NLP) analysis enables the quantitative measurement of speech and language alterations in schizophrenia that can provide a more nuanced characterization of speech-based symptom expression and identify objective “digital biomarkers” of underlying illness processes.
This approach begins with obtaining patient speech recordings, either from clinical interviews or often from brief, speech-focused tasks, such as describing the contents of a picture or answering open-ended questions as a “verbal journal.” The speech recording must then be transcribed to text, either using automated speech recognition tools or manual transcription. The speech recording undergoes signal analysis to extract acoustic properties characterizing how the voice sounds (eg, in terms of pitch, volume, and rate), while NLP algorithms are applied to the speech transcript to quantify the content, meaning, and level of organization of the patient’s speech. The output of this analysis is a multitude of speech variables that objectively and reliably characterize the patient’s speech and language, which can be applied to clinical contexts.
To date, research applying NLP to schizophrenia spectrum disorders has focused on the following 4 main areas of clinical application:
- Diagnostic Classification: eg, distinguishing between individuals with schizophrenia vs. healthy controls and assisting in differential diagnosis;
- Measuring symptom severity: eg, formal thought disorder, positive symptoms, negative symptoms;
- Predicting illness onset: eg, predicting clinical high-risk youth who will transition to psychosis; and
- Informing prognosis and treatment outcomes: eg, predicting individuals who will receive a schizophrenia diagnosis following a first psychotic episode.
Thistle, PCN: What key practical implications were discussed in your session for clinicians treating schizophrenia?
Dr Spilka and Dr Tang: Speech and language assessment provides clinically useful information to understand and treat patients with schizophrenia spectrum disorders. Speech alterations in schizophrenia reflect both the symptoms of the disorder (e.g., alogia), as well as underlying illness factors (e.g., affective states, thought process and level of organization, cognitive functioning).
Thistle, PCN: What are the primary challenges for clinicians conducting speech evaluation in patients with schizophrenia spectrum disorders?
Dr Spilka and Dr Tang: Speech is a core part of the Mental Status Exam, but clinical evaluation of speech disturbances is nuanced, subjective, and requires extensive training. Computerized speech assessment and analysis can overcome these limitations to provide low-burden, objective, and comprehensive measurement of speech alterations in schizophrenia to complement clinician ratings.
Thistle, PCN: How should clinicians be integrating natural language processing (NLP) into their existing care practices/approaches?
Dr Spilka and Dr Tang: At present, clinicians interested in computational speech analysis will likely need to collaborate with researchers employing these methods. However, the assessment of speech itself is low-burden, and several brief, speech-focused assessment options exist that are mobile phone or tablet-based and are therefore suitable for remote, high-frequency assessment and monitoring.
Thistle, PCN: What are the limitations of NLP? What should clinicians be wary or mindful of when using it as a tool?
Dr Spilka and Dr Tang: NLP and computational methods have shown promise for identifying objective markers for predicting, diagnosing, and monitoring schizophrenia spectrum disorders.
However, the following barriers must be addressed before these approaches can be integrated into clinical practice:
- We need to determine the computational speech features that generalize across diverse samples, particularly with respect to sociocultural and linguistic diversity, to ensure that NLP markers will have high fidelity in the clinic;
- The variety of methods to elicit and analyze speech currently employed must be compared and evaluated to determine the most sensitive approaches to carry forward for a standardized approach to deploy in clinical practice;
- The sensitivity and specificity of speech markers in psychosis continue to be examined to ensure that these markers reflect the clinical variable of interest (eg, making sure that speech markers of negative symptom severity are not confounded by comorbid medical symptoms); and
- The careful consideration of ethical issues in the context of digital speech measures, including protecting patient privacy and confidentiality, and guarding against the risk of bias in NLP analysis (e.g., biases based on sociocultural factors) to minimize the risk of harm and promote equitable healthcare.
Thistle, PCN: What misconceptions on this topic would you like to clear up?
Dr Spilka and Dr Tang: NLP uses computational tools to describe different properties of speech and language, and when combined with artificial intelligence (AI) or machine learning (ML), can help interpret, learn, and make decisions from speech assessment data. However, these tools currently do not replace expert clinician judgment; rather, they can be used to augment traditional clinical evaluation and decision-making.
Michael J. Spilka, PhD, is a scientist at Winterlight Labs in Toronto, Canada, and earned a PhD in Clinical Psychology from the University of Calgary.
Sunny Tang, MD is is an assistant professor of psychiatry at the Feinstein Institutes and the Donald and Barbara Zucker School of Medicine at Hofstra/Northwell. Dr Tang is a board-certified psychiatrist who earned her medical degree at SUNY Downstate College of Medicine before completing psychiatry residency training at the University of Pennsylvania.