ADVERTISEMENT
Artificial Intelligence, Machine Learning Show Promise for Recovery, Behavioral Health
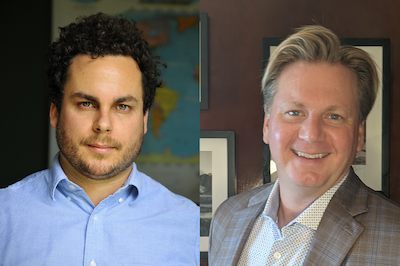
The growth of artificial intelligence (AI) solutions in healthcare is expanding rapidly. Such growth is likely slowed only by a lack of qualified data scientists to meet present demand. AI technologies are based on algorithms, which are digitally stored and implemented instructions on how to compare and divide sets of data. The 1970s saw the emergence of AI in biomedical fields and advances, particularly in neuroscience, as computers were able to translate brain activity into interactive sets of data.
Data science, machine learning and AI are standard technologies today in medical and health fields. They are making headway in oncology, neuroscience and vaccinology. Recent decades have also witnessed AI advances in behavioral, physical, and mental health.
Several AI-based wearable options are presently available. They can make accurate predictions about present states of stress and anxiety based upon measures of heart rate, breathing, and movement. In addition to wearables, machine learning algorithms have been developed to harvest data from phone usage, including swiping, typing, and other movement patterns. The integration of that data with healthcare providers may represent the next major advancement in healthcare. For example, Apple recently announced the ability to securely share health data collected through an iPhone or Apple Watch with trusted partners such as physicians or other caregivers.
At its core, a “data-driven” approach is one that not only remains faithful to measurable evidence, but also marshals the quantified nature of datasets to find new solutions. For example, a conventional provider might work with a patient struggling with anxiety disorder by observing patterns of behavior, soliciting history of trauma and following trial-confirmed treatment protocols. One of the most promising applications of AI to such a patient is its ability to discover correlations between patterns that would otherwise be invisible to the provider, thereby enabling more individualized treatment.
So where is this data, and how do scientists get it? Research published by The National Center for Telehealth & Technology found smartphones provide a ubiquitous, though controversial, solution. There are now hundreds of self-assessment applications able to track users’ sleep, breathing, oxygen levels, movement, stress, and mood. Smartphones store information beyond those elected self-assessments with the ability to inform mental and behavioral health through secondary applications that apply algorithms to data. Examples are the way one communicates through text, time of use, app selection, location, and analysis of audio, visual, and video content. Pattern recognition algorithms can predict the likelihood of issues such as suicidal ideation even from within a single application such as Facebook. Whether correlations exist between this information and the direct information about sleep, movement, and mood is yet another application of machine learning.
The most direct integration of biomedical and behavioral AI in recovery treatment is through already existing mental health inroads because substance use disorder is so often compounded with other issues such as trauma, anxiety, depression, and social dysfunction. In one instance, researchers applied pattern recognition to social media addiction-related activity to predict relapse probability. In the realm of wearables and environmental monitoring, some recovery treatment providers are prescribing the passive sleep monitor EarlySense to predict relapse risks. It can record vitals, sleep, and motion, including restlessness, seizures, and falls.
Much of the most advanced AI-powered work in recovery research focuses on the contexts surrounding behavioral disorders such as addiction. Social contexts and relationships frequently feature among the highest predictors in data analytics for mental and behavioral wellness. Scientists at the University of Southern California created an algorithm to compare the social behaviors of young people with substance use disorders among their peers. It then predicts more effective peer groups based on that information. In addition, geofencing applications have been developed to alert individuals with SUD and their social supports when they are near specific locations designated as high risk to making poor health decisions.
Data-driven smartphone applications have also emerged in the recovery field. Sometimes the simplest solution is optimal, as with Sober Grid, an application that connects those in recovery from alcohol addiction to form support groups. Triggr is an application combining self-assessment, social networking, and virtual treatment for individuals in recovery, and capitalizes on the power of remote support for people who might otherwise avoid anonymous groups. Addicaid goes a step further through automated micro-interventions such as alerting a provider if a user enters a compromising location.
As in the last 2 instances, the most effective treatments combine deep data analytics with irreplaceably human applications. Relationships, personal history, trauma, sleep, and conversation form the foundations for many of the most popular and promising AI applications in mental and behavioral healthcare, including recovery.
Matthew J. Smith, PhD, is director of human sciences at Alter Health Group and Loren Martin, PhD, is chief science officer at Alter Health Group and professor of clinical psychology at Azusa Pacific University.