Principal Component Analysis Identifies Hidden Electrogram Features That Predict Outcomes Atrial Fibrillation Ablation
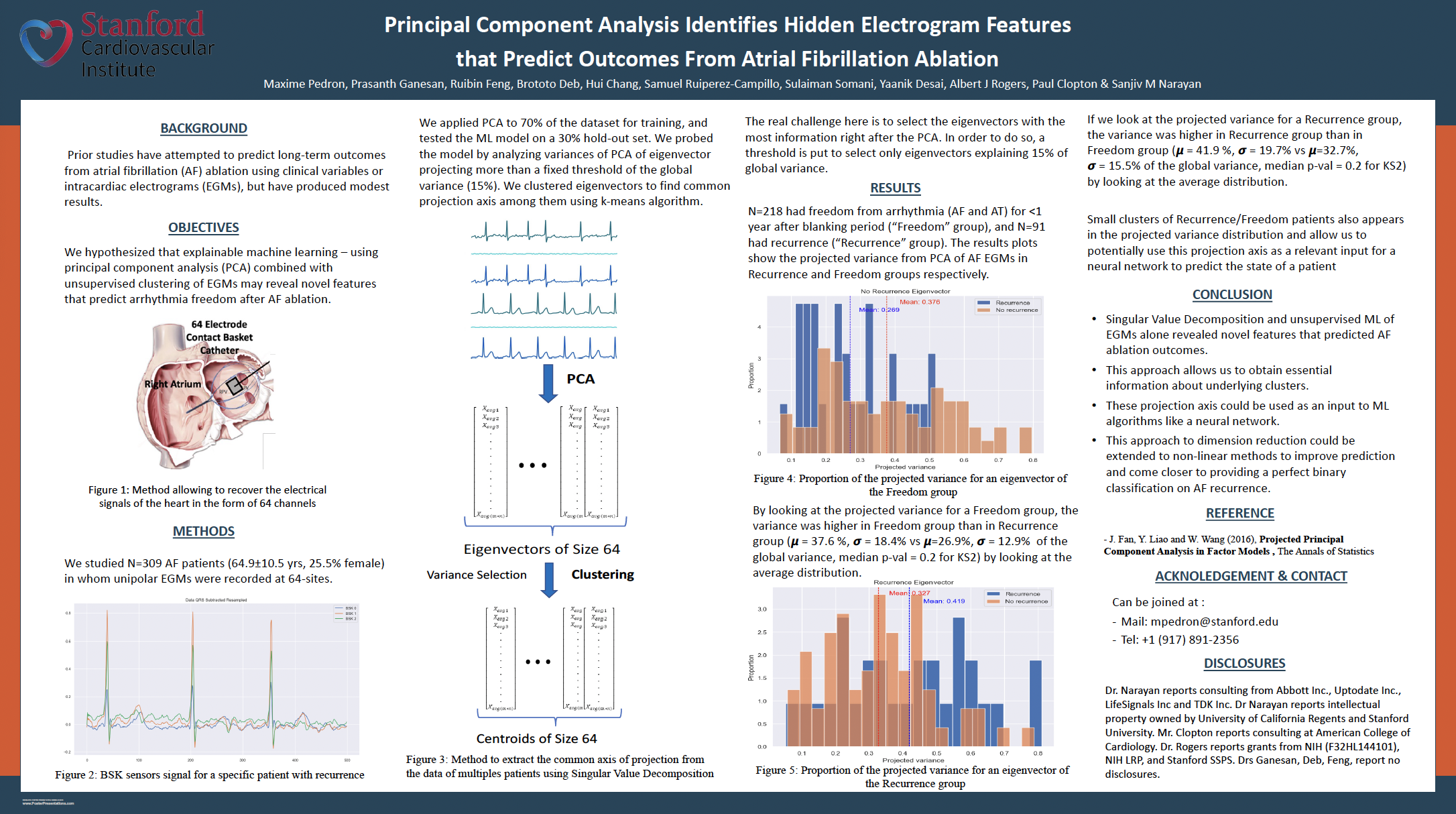
Purpose: Background
Prior studies have attempted to predict long-term outcomes from atrial fibrillation (AF) ablation using clinical variables or intracardiac electrograms (EGMs), but have produced modest results.
Hypothesis
We hypothesized that explainable machine learning – using principal component analysis (PCA) combined with unsupervised clustering of EGMs may reveal novel features that predict arrhythmia freedom after AF ablation.
Material and Methods: We studied N=309 AF patients (64.9±10.5 yrs, 25.5% female) in whom unipolar EGMs were recorded at 64-sites. We applied PCA to 70% of the dataset for training, and tested the ML model on a 30% hold-out set. We probed the model by analyzing variances of PCA of eigenvector projecting more than a fixed threshold of the global variance (15%). We clustered eigenvectors to find common projection axis among them using k-means algorithm.
Results: N=218 had freedom from arrhythmia (AF and AT) for < 1 year after blanking period (“Freedom” group), and N=91 had recurrence (“Recurrence” group). The results plots show the projected variance from PCA of AF EGMs in Recurrence and Freedom groups respectively. Variance was higher in Recurrence group than in Freedom group (1.51 ± 0.7% vs 1.3 ±0.3% of the global variance) by looking at the average distribution. Small cluster of Recurrence/Freedom patient also appears in the projected variance distribution and allows us to potentially use this projection axis as a relevant input for a neural network to predict the state of a patient
Conclusions: PCA and unsupervised ML revealed novel features that predicted AF ablation outcomes. This approach to dimension reduction could be extended to non-linear methods or other techniques to identify features for improved prediction.