Machine Learning of AF Electrograms and Clinical Features Predict Acute Termination of Atrial Fibrillation by Ablation
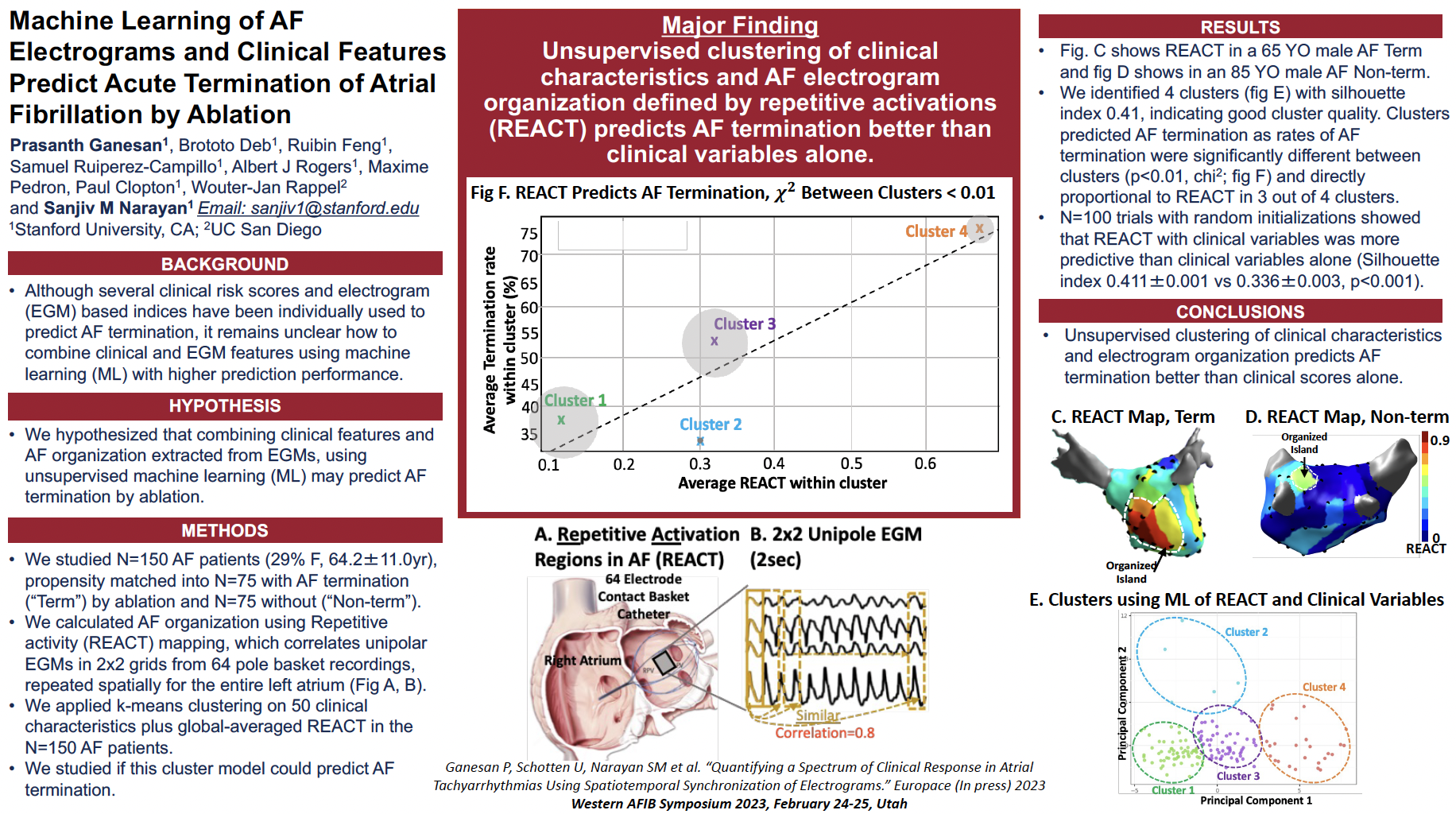
Purpose: Several clinical risk scores have been used to predict outcomes from ablation of atrial fibrillation (AF). Several electrogram-based indices have also been used, namely, dominant frequency gradients, consistent repetitive electrograms (EGMs), and disorganized EGMs. It remains unclear how to combine clinical features and EGM features to predict AF termination by ablation. We hypothesized that combining clinical features and AF organization feature extracted from EGMs using unsupervised machine learning (ML) may predict AF termination by ablation.
Material and Methods: We studied N=150 AF patients (29% women, 64.2±11.0 years), propensity matched into N=75 with AF termination (“Term”) and N=75 without (“Non-term”) by pulmonary vein isolation and targeted ablation. We calculated AF organization using Repetitive activity (REACT) mapping, which correlates unipolar EGMs in 2x2 grids from 64 pole basket recordings over 4 sec, repeated spatially for the entire left atrium. We applied k-means clustering on 50 clinical characteristics plus global-averaged REACT in the N=150 AF patients. We studied if this cluster model could predict AF termination.
Results: REACT values were higher in AF patients with successful termination than patients without termination (0.37±0.22 vs 0.22±0.18, p< 0.001). We identified 4 clusters with silhouette index 0.41, indicating good cluster quality. Clusters predicted AF termination as rates of AF termination were significantly different between clusters (p < 0.01, Chi sq.) and directly proportional to REACT in 3 out of 4 clusters. N=100 trials with random initializations showed that REACT with clinical variables was more predictive than clinical variables alone (Silhouette index 0.411±0.001 vs 0.336±0.003, p< 0.001).
Conclusions: Unsupervised clustering of clinical characteristics and electrogram organization predicts AF termination better than clinical risk scores alone. Future studies should investigate supervised machine learning of clinical and electrogram features for predicting ablation success.